
Timeseries, Transformers, and Two Cultures
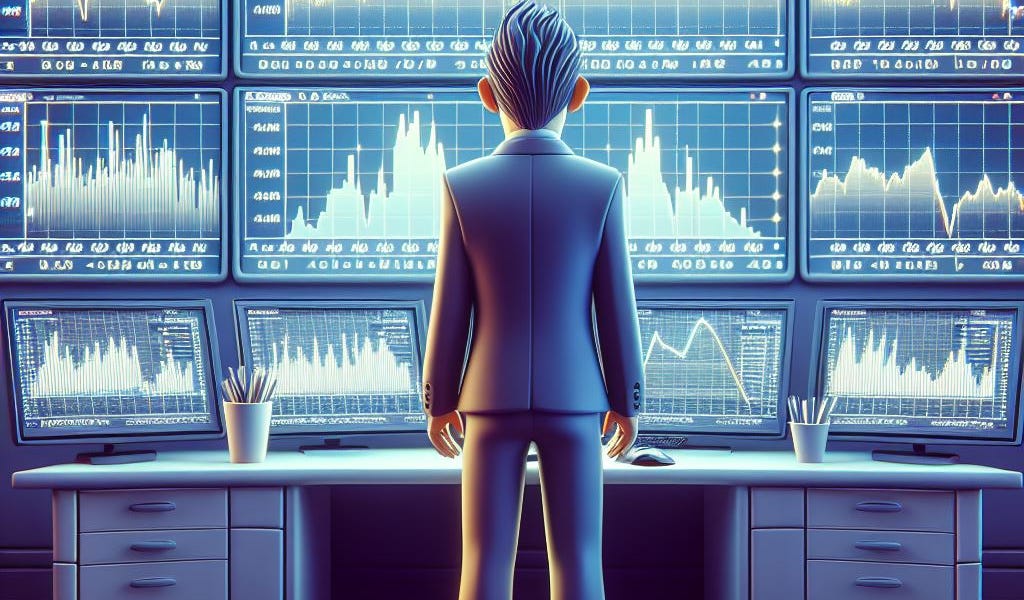
๐ Abstract
The article discusses the two cultures in statistical learning - one that assumes the data is generated by a stochastic process that can be modeled, and another that treats the data generating process as unknown but uses algorithms to make predictions. It examines how transformer-based neural networks have performed in forecasting chaotic systems like geopolitics, financial markets, and weather, and presents three hypotheses on whether and how these models can overcome their relative underperformance in this domain.
๐ Q&A
[01] Timeseries, Transformers, and Two Cultures
1. What are the two cultures in statistics described in the article?
- The first culture assumes the data is generated by a stochastic process that can be modeled, while the second culture treats the data generating process as unknown but uses algorithms to make predictions.
2. How has the dominance of the two cultures shifted over time?
- The first culture was more dominant when the paper by Leo Breiman was written, but the second culture has become more dominant as large datasets and cheap compute made black box approaches more practical.
3. How have neural networks and transformers performed in forecasting chaotic systems compared to other methods?
- Neural networks and transformers have not yielded similar advances in forecasting chaotic systems like geopolitics, financial markets, and weather, compared to their success in other domains. Basic benchmarks show transformer-based models underperforming ensembles of standard econometric forecasting models.
[02] Hypotheses on Transformer-Based Models and Chaotic Timeseries Forecasting
1. What are the three hypotheses presented in the article on whether and how transformer-based models can overcome their underperformance in chaotic system forecasting?
- The three hypotheses are:
- Transformer-based models will eventually solve this problem as they continue to improve.
- There is not enough data, especially observations of events, due to the long timescales of chaotic systems.
- Transformer-based models will not directly solve chaotic forecasting, but will help identify and apply specialized statistical methods from the first culture.
2. What is the reasoning behind the second hypothesis on lack of data?
- The argument is that certain stochastic processes across regimes take an extremely long time to observe, such as only around 8 recessions since 1945. This makes it difficult to capture sufficient observations to train models. Additionally, the problem of reflexivity is mentioned, where the act of successfully forecasting certain chaotic systems can change the system itself.
3. How does the third hypothesis suggest transformer-based models could contribute to chaotic system forecasting?
- The third hypothesis proposes that transformer-based models may not directly solve chaotic forecasting, but could serve as "co-pilots" or "auto-pilots" to identify opportunities to apply highly specialized but non-transformer based models from the first culture of statistics, which are often difficult to parameterize and require domain knowledge.