
Why transformative artificial intelligence is really, really hard to achieve
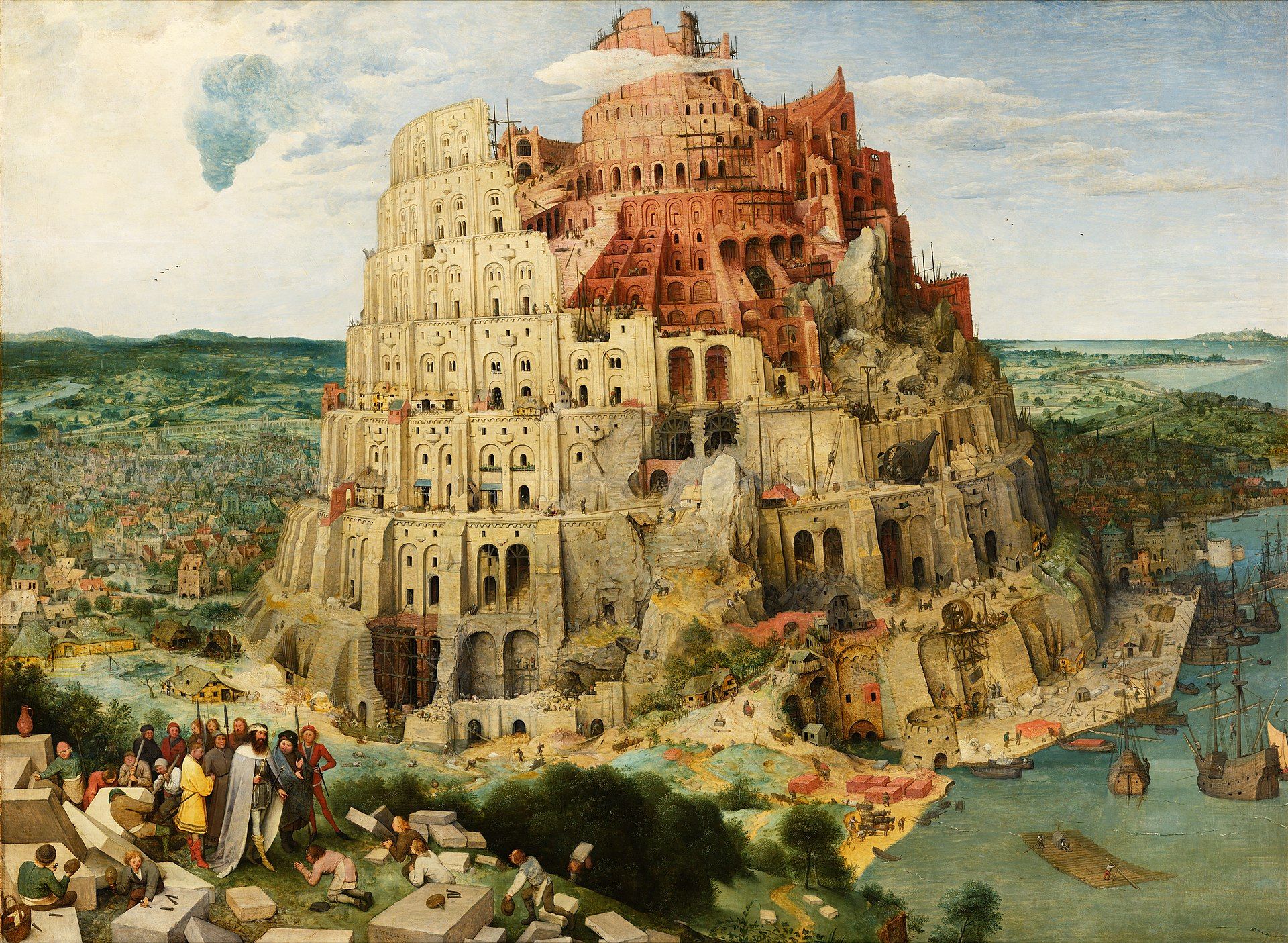
๐ Abstract
The article discusses the potential for transformative AI to drive rapid economic growth, and the various hurdles and challenges that may prevent this from happening. It argues that while AI has the potential to be a powerful technology, it faces significant obstacles in automating all essential economic sectors and steps of the innovation process. The article draws on economic theories and historical examples to suggest that AI may be more akin to a "prosaic" technology like the steam engine, rather than a technology that will lead to an "intelligence explosion" and exponential growth.
๐ Q&A
[01] Visions of Transformative AI
1. What is the goal of many top AI labs when it comes to AI performance? Many top AI labs explicitly have the goal of developing AI systems that are as good as or better than humans at all economically valuable tasks.
2. What is the risk in measuring AI's performance on a predetermined set of tasks? Measuring AI's performance on a predetermined set of tasks is risky, as real-world impact may require doing tasks that we are not even aware of.
3. How do the authors define "transformative AI" in the context of this essay? The authors define transformative AI in terms of its observed economic impact, specifically its ability to drive productivity growth that could break the 3% per capita per year threshold and potentially accelerate.
[02] Bottlenecks to Transformative AI
1. What is Baumol's insight about productivity growth? Baumol noted that productivity growth that is unbalanced across sectors may be constrained by the weakest sector, as sectors that are hard to improve in efficiency become relatively more valuable.
2. How have historical productivity gains been affected by uneven innovation across sectors? Historical data shows that uneven innovation across sectors, with technology boosting productivity in some sectors but not others, has slowed down aggregate productivity growth.
3. What does the concept of "bottlenecking" suggest about the hurdles to achieving transformative AI? The concept of bottlenecking suggests that AI must transform all essential economic sectors and steps of the innovation process, not just some of them, in order to achieve transformative growth. Otherwise, the chance that AI should be viewed as similar to past inventions goes up.
[03] Open Research Problems for Transformative AI
1. What are some examples of open research problems relevant to transformative AI? Examples include learning causal models, the importance of embodiment, and the need for efficient systems with strong generalization power.
2. What do the authors suggest about the difficulty of these open problems? The authors suggest that even simple indicator tasks like walking and wriggling (neurological simulation of worms) remain intractable, which must be taken as a "bearish signal" for transformative AI.
3. How do the authors view the potential for current AI methods to be sufficient for achieving transformative AI? The authors suggest that the limits of current AI methods may soon be upon us, and that we may have exhausted the low-hanging fruit in hardware optimization and are now entering an era of deceleration.
[04] The Role of Humans in AI Development
1. How do the authors view the role of human feedback and input in AI development? The authors argue that insofar as AI development requires human input, humans will constrain productivity, as their expertise and creative spark become more valuable.
2. What is the significance of tacit knowledge for transformative AI? The authors suggest that a large share of human knowledge is tacit, unrecorded, and diffuse, which may make it difficult for AI to surpass human performance in many important settings.
3. How do the authors view the potential for AI to automate the process of innovation itself? The authors are skeptical that AI can automate the entire innovation process, as some problems may be too hard for brains alone, whether biological or artificial.
[05] Historical Lessons and Limitations of AI
1. What lessons do the authors draw from the history of economic transformation? The authors argue that history is one of contingency, where many factors must come together for transformation to occur, rather than a single factor outweighing all else.
2. How do the authors view the potential for social and political barriers to slow down or halt the adoption of AI technology? The authors provide examples of how social and political barriers have slowed down or halted the adoption of other transformative technologies, and suggest the same could happen with AI.
3. What are the authors' views on the potential for AI to decrease productivity in certain domains? The authors suggest that some current use cases of AI, such as recommender systems for social media, may actually decrease productivity rather than increase it.
[06] Conclusion
1. What is the authors' overall perspective on the potential for transformative AI? The authors believe that the case for AI being an invention elevated far above the rest is not closed, and that AI may end up more like a "prosaic" powerful technology, similar to the steam engine, rather than leading to an "intelligence explosion" and exponential growth.
2. What do the authors recommend in terms of investment and focus when it comes to AI development? The authors recommend investing in the hardest problems across innovation and society, rather than jumping to the most flashy recent developments in AI, and tracking progress across many domains of innovation, not just AI's star subfields.