
AI and the "Why Now" of Data DAOs
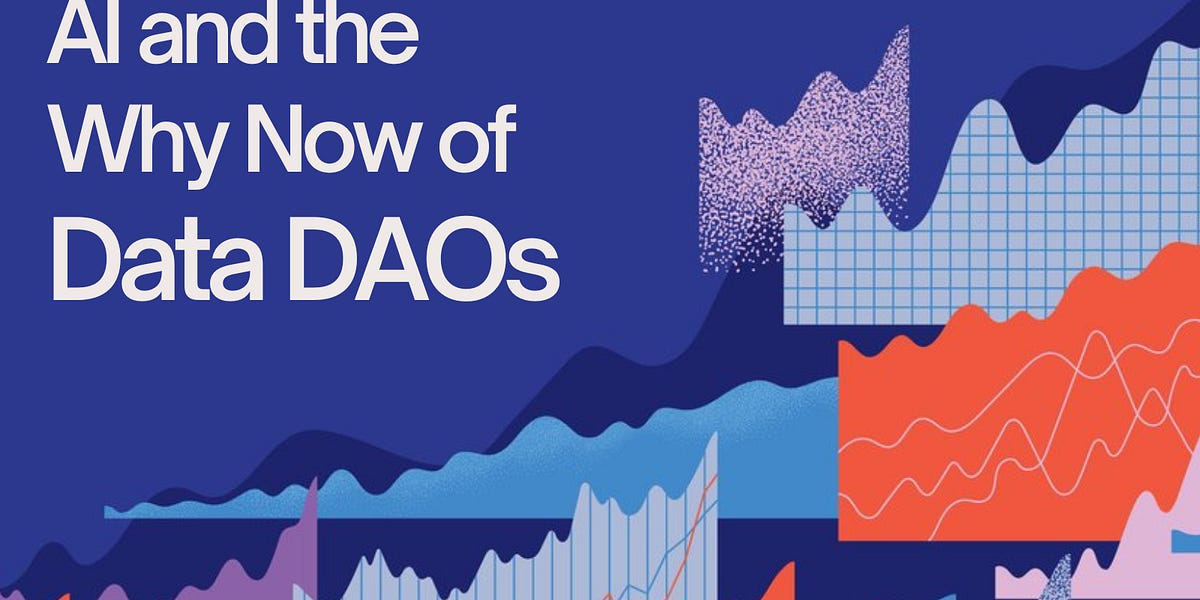
๐ Abstract
The article discusses the potential of data DAOs (decentralized autonomous organizations) to accelerate AI development by addressing the limitations of current data aggregation methods. It explores how data DAOs could help generate new datasets, economically reward contributors, and overcome the "data wall" that limits further algorithmic improvements in AI.
๐ Q&A
[01] Recent High-Profile Data Licensing Deals and the Need for High-Quality Data in AI
1. What are the key points made about recent high-profile data licensing deals and the need for high-quality data in AI?
- Recent high-profile data licensing deals, such as those between OpenAI and News Corp and Reddit, underscore the need for high-quality data in AI.
- Frontier models are already trained on much of the internet, including data from sources like Common Crawl, which contains over 100 trillion tokens.
- Expanding and enhancing the data that AI models can train on is an avenue for further improvement.
[02] The Idea of Data DAOs
1. What is the idea of data DAOs, and why is the rapid advancement of AI a catalyst for this?
- The idea of data DAOs, or collectives of individuals who create, organize, and govern data, has been discussed in the crypto space in recent years.
- The rapid advancement of AI is a catalyst for a new "why now?" of data DAOs, as AI models are increasingly reliant on high-quality data for training.
[03] Limitations of Current Data Aggregation Approaches
1. What are the limitations of the current approaches to data aggregation for AI training?
- The current approaches to data aggregation, such as partnerships and data scraping, have limitations in terms of what data they can collect and how they collect it.
- AI development is bottlenecked by data quality and quantity, and the "data wall" limits further algorithmic improvements.
- There are vast quantities of private data that are out of reach for AI training today, such as enterprise data, personal health data, and private messages.
- Under the existing paradigm, companies that aggregate data capture the majority of the value, while end users who generate the data do not see any economic benefit.
[04] How Data DAOs Could Address Gaps in the Current Data Landscape
1. What are some of the gaps in the current data landscape that data DAOs could potentially address?
- In the decentralized physical infrastructure (DEPIN) world, networks like Hivemapper aim to collect the world's freshest global map data by incentivizing dashcam owners and users to contribute data, with revenues accruing back to contributors.
- Data DAOs could bring structure and incentives to biohacking efforts by organizing participants around common experiments and collecting results, with revenue passed back to the participants.
- Data DAOs could help source and incentivize expert participation in fine-tuning AI models with RLHF (reinforcement learning with human feedback) through token rewards.
- Data DAOs could enable willing participants to upload and monetize their private data, such as Reddit comments and posts, in a privacy-preserving way, with token incentives allowing users to earn based on the value created by the AI models trained on their data.
[05] Considerations and Challenges for Data DAOs
1. What are some of the key considerations and challenges for data DAOs?
- Token incentives could skew the participant base and the type of data being contributed, as extrinsic incentives can alter user behavior.
- There is a risk of participants submitting low-quality or fabricated data to maximize their earnings, which could undermine the value of the dataset.
- Establishing robust mechanisms to verify the authenticity and accuracy of data is crucial to prevent fraudulent submissions or Sybil attacks.
- Data DAOs need to ensure that the data they collect is truly incremental and additive to the existing data available on the open web, and that the revenue opportunity is large enough to incentivize the quantity and quality of data needed.
- Data DAOs need to identify and validate their end demand, ensuring that there is a stable and diverse customer base willing to pay for the data.