
The Perils of Moneyballing Everything
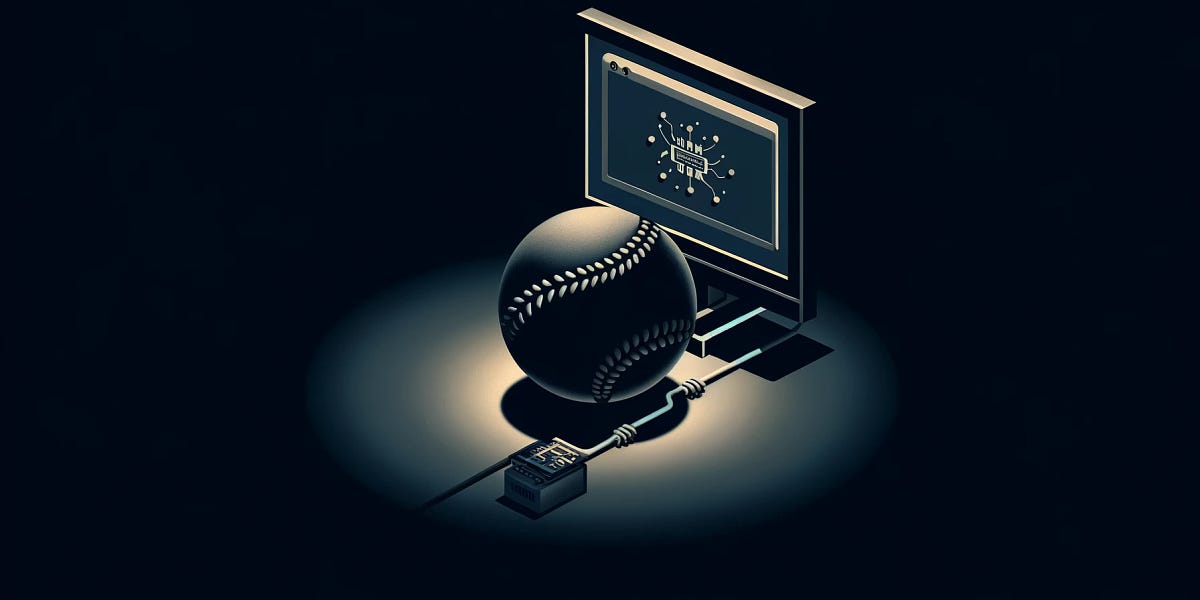
๐ Abstract
The article discusses the perils of over-relying on data analytics and treating complex "mysteries" as if they were simple "puzzles". It highlights the distinction between puzzles (well-defined problems with clear rules and outcomes) and mysteries (loosely defined problems with radically uncertain outcomes), and cautions against applying Moneyball-style data analytics to solve Calvinball-like problems. The article argues that while data analytics can be powerful in solving puzzles, they can create catastrophic risks when misapplied to complex, ever-changing systems. It emphasizes the importance of recognizing the difference between puzzles and mysteries, and adopting appropriate strategies for each - optimization for puzzles, and resilience and experimentation for mysteries.
๐ Q&A
[01] Moneyball and the Wizardry of Analytics
1. What is the key distinction between puzzles and mysteries, as described in the article?
- Puzzles are well-defined problems with clear rules and a straightforward set of possible outcomes, where past patterns offer an accurate guide to future outcomes.
- Mysteries are loosely defined problems with radically uncertain outcomes, where the past offers no credible guide to future outcomes.
2. How did the Moneyball approach revolutionize sports analytics?
- The Moneyball approach, pioneered by the Oakland Athletics under Billy Beane, used sophisticated data analytics to spot value where others overlooked it, realizing that understanding a team, like understanding any system, often requires more than just analyzing constituent parts.
- This systems-based, data-driven approach transformed sports, making statistics central to modern sports analysis and team management.
3. How did the "Moneyball" concept get misapplied in the business world?
- The article criticizes the "LinkedIn bros" who claimed that "everything should be moneyballed" and that deploying data analytics could magically fix any problem, despite the world not being actually fixed two decades after Moneyball.
[02] Treating Mysteries as Puzzles Will Destroy Us
1. What is the key distinction between puzzles and mysteries, as described in the article?
- Puzzles are easy, well-defined problems where past patterns offer an accurate guide to future outcomes.
- Mysteries are hard, loosely defined problems with radically uncertain outcomes, where the past offers no credible guide to future.
2. How does the article caution against treating mysteries as puzzles?
- The article warns that treating mysteries as puzzles and deploying data analytics as the sole tool for decision-making in radically uncertain environments can lead to "human-engineered catastrophe borne from the hubris of misapplied Big Data".
- It argues that powerful tools used incorrectly are dangerous, as they can make us behave with arrogant certainty in the face of radical uncertainty.
3. What is the key difference between closed systems and open systems, as described in the article?
- Closed systems have stable cause and effect relationships, a finite set of inputs, and a constrained set of outputs.
- Open systems have ever-changing cause and effect relationships, far more chaos than any model can process, and where just about anything can happen.
[03] The Optimization / Resilience Trade-off
1. What is the key trade-off described in the article when dealing with puzzles vs. mysteries?
- In puzzles (the stable world of closed systems), there is limited downside to ever-greater analytics and optimization.
- In mysteries (the open, complex systems we inhabit), there is a trade-off between optimization and resilience, where focusing too much on optimization courts disaster.
2. What are the key lessons the article provides for dealing with mysteries?
- Use data analytics only to optimize when solving puzzles, not mysteries.
- If facing a mystery, dial down efforts to optimize, rely less on flawed data models, and focus instead on resilience and experimentation.
- Avoid the mistake of optimizing for the wrong metric, even if you're getting ever-better at it.
[04] The McNamara Fallacy, the Surefire Mediocre, and the Emptiness of a Lifehack Lifestyle
1. What is the McNamara Fallacy, and how did it manifest in the Vietnam War?
- The McNamara Fallacy is the false notion that every problem can be analyzed and solved exclusively with quantitative data and better metrics.
- In Vietnam, McNamara fetishized the "body count" metric, ignoring difficult-to-measure aspects that decide wars, such as the hearts and minds of the local people.
2. How did Moneyballing lead to the "surefire mediocre" in creative realms?
- When applied incorrectly, data analytics can lead to convergence toward banal blockbusters, McMansion architecture, and simply replicating what worked previously, stifling creativity and cultural vibrancy.
3. What is the article's warning about optimizing our lives through data and "lifehacks"?
- The article cautions against devoting our extraordinary human consciousness solely to a "Lifehack Lifestyle" of unquenchable thirst for optimization, at the expense of taming the wildness that makes us human.