
Compute Moonshots: Harnessing New Physics
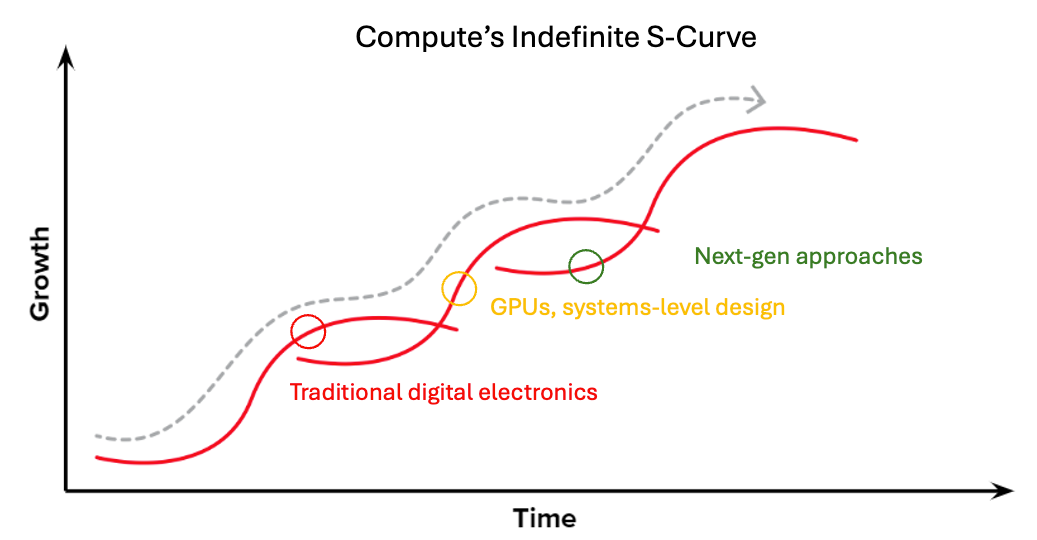
๐ Abstract
The article discusses the challenges facing the digital computing domain and the need for new approaches that harness different materials and physics to address the growing demand for compute power, particularly driven by AI and HPC workloads. It explores various alternative computing paradigms, including photonics, thermodynamics/stochastic computing, quantum computing, in-memory computing, and in-sensor computing, among others. The article highlights the potential of these approaches to provide significant efficiency gains over traditional digital electronics, while also acknowledging the technical challenges and barriers to their widespread adoption.
๐ Q&A
[01] The digital computing domain
1. What are the key challenges facing the digital computing domain?
- The physics of traditional digital electronics are enforcing fundamental limits on their speed and efficiency, such as slow and resistive electrons, the need for continual energy to store data, and the von Neumann bottleneck.
- The physical limits of placing gates closer together on silicon have been reached, leading to plateaus in clock speed performance and energy density/efficiency.
- The increasing complexity and costs of making chips have resulted in diminishing returns.
- The density of memory technology has slowed, even as AI places exponentially growing demands on it.
2. What is the status of Moore's Law?
- While Moore's Law is not dead, the industry will need to overcome increasingly difficult physics challenges to continue the trend of improving digital compute performance.
- Each successive engineering miracle needed to overcome the ever-gnarlier physics of digital approaches will increasingly tilt the incentive towards finding systems with entirely different physics.
3. What is the potential for efficiency gains compared to the brain?
- Cutting-edge digital processors are still 1 million times more energy inefficient than the brain, suggesting significant room for progress.
[02] Alternative computing approaches
1. What are some of the alternative computing approaches discussed in the article?
- Photonics
- Thermodynamics/stochastic computing
- Quantum computing
- In-memory computing
- In-sensor computing
- Reversible computing
- Magnetics, magnons, and spintronics
- Brain organoid-based computing
- Information-secure architectures for AI servers
2. What are the key advantages and challenges of these alternative approaches?
- Most of these approaches are analog, which can provide significant efficiency gains but also face challenges such as lack of CAD software, programming tools, error correction, and device-device variability.
- Integrating analog and digital components can negate some of the performance advantages and create design complexities.
- However, as AI moves towards lower precision, the lower accuracy of analog circuits may become less problematic.
3. What are the potential paths for startups to compete with incumbents like Nvidia?
- Startups can pursue highly efficient, "moonshot" approaches that have the potential for 100x efficiency improvements over current state-of-the-art chips.
- Startups can also take a software-defined, capital-light approach, or partner with Nvidia's competitors to embed their alternative physics approach.
- Overcoming Nvidia's software moat is a significant challenge, requiring either vertical integration or close collaboration with Nvidia's competitors.
[03] Industry dynamics and outlook
1. How might the industry structure evolve with the emergence of specialized chips?
- The market may accept lower generality in exchange for step-function performance improvements as model architectures standardize and use cases mature.
- This could lead to a shift from an oligopoly to an industry structure with extreme power laws, where chips custom-built for specific tasks and use cases thrive.
- However, the industry may continue to value flexibility and the ability to respond to whatever may be on the horizon, which could limit the viability of highly specialized chips.
2. What potential inflection points could drive the adoption of alternative physics approaches?
- The ever-increasing energy demands of AI and other compute-intensive applications, such as robots and autonomous vehicles, may place a hard ceiling on the continued scaling of traditional digital compute, creating an opportunity for alternative approaches.
- Political and societal upheaval over the continent-scale, exponentially-growing energy demand of AI could also drive the adoption of more energy-efficient computing solutions.