
AGI Safety and Alignment at Google DeepMind: A Summary of Recent Work — AI Alignment Forum
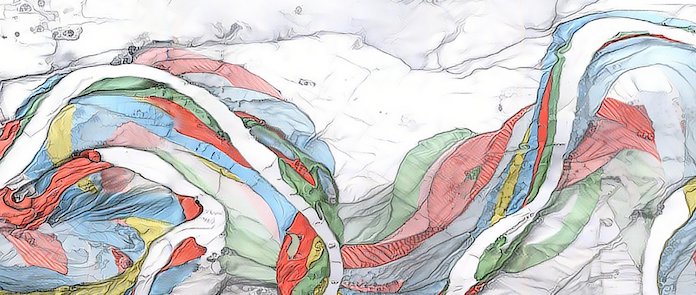
🌈 Abstract
The document provides an update on the work being done by the AGI Safety & Alignment team at Google DeepMind, covering their key focus areas, recent publications, and ongoing collaborations.
🙋 Q&A
[01] Overview of the AGI Safety & Alignment team
1. What are the main focus areas of the AGI Safety & Alignment team?
- The team's main bets for the past 1.5 years have been:
- Amplified oversight, to enable the right learning signal for aligning models and preventing catastrophic risks
- Frontier safety, to analyze whether models are capable of posing catastrophic risks
- (Mechanistic) interpretability, as a potential enabler for both frontier safety and alignment goals
2. What is the mission of the Frontier Safety team?
- The mission of the Frontier Safety team is to ensure safety from extreme harms by anticipating, evaluating, and helping Google prepare for powerful capabilities in frontier models.
3. What is the Frontier Safety Framework (FSF) and how does it differ from other approaches?
- The FSF follows the approach of responsible capability scaling, similar to Anthropic's Responsible Scaling Policy and OpenAI's Preparedness Framework.
- The key difference is that the FSF applies to Google, as there are many different frontier LLM deployments across Google, rather than just a single chatbot and API.
4. What are the team's priorities as they pilot the Frontier Safety Framework?
- A key focus is on mapping between the critical capability levels (CCLs) and the mitigations that would be taken.
[02] Mechanistic Interpretability
1. What are the team's recent advancements in mechanistic interpretability?
- The team has focused deeply on Sparse AutoEncoders (SAEs), releasing new architectures like Gated SAEs and JumpReLU SAEs that substantially improved the Pareto frontier of reconstruction loss vs sparsity.
- They have also released Gemma Scope, an open, comprehensive suite of SAEs for the Gemma 2 2B and 9B models, to enable more ambitious interpretability research outside of industry labs.
[03] Amplified Oversight
1. What are the key challenges the team has encountered in their amplified oversight work?
- In inference-only experiments, they found that debate performs significantly worse than giving the judge access to the full information on tasks with information asymmetry.
- They also found that weak judge models with access to debates don't outperform weak judge models without debate on tasks without information asymmetry.
- The team believes these issues occur because the models are not very good at judging debates, and their current work is focused on training their LLM judges to be better proxies of human judges.
[04] Other Research Areas
1. What are some of the other research areas the team has explored?
- Causal incentives and how understanding causal incentives can contribute to designing safe AI systems
- Challenges with unsupervised LLM knowledge discovery, demonstrating the existence of many "truth-like" features that can trick unsupervised knowledge discovery approaches
- Explaining grokking through circuit efficiency, to better understand training dynamics and their implications for safety
2. How does the team collaborate with other teams and external researchers?
- Within Google, the team collaborates with the Ethics and Responsibility teams on issues around value alignment, manipulation, and persuasion.
- Externally, the team collaborates with members of the causal incentives working group and contributes to benchmarks and evaluations of potentially unsafe behavior.
- The team also mentors MATS scholars on a wide range of topics related to their work.
[05] Revising the High-Level Approach
1. What is the team's current focus on revising their high-level approach to technical AGI safety?
- The team is mapping out a logical structure for technical misalignment risk, and using it to prioritize their research to better cover the set of challenges they need to overcome.
- This includes addressing areas like distribution shift, where the AI system could behave in ways that amplified oversight wouldn't endorse, and investing in mitigations such as adversarial training, uncertainty estimation, and monitoring.