
A physicists’ guide to the ethics of artificial intelligence
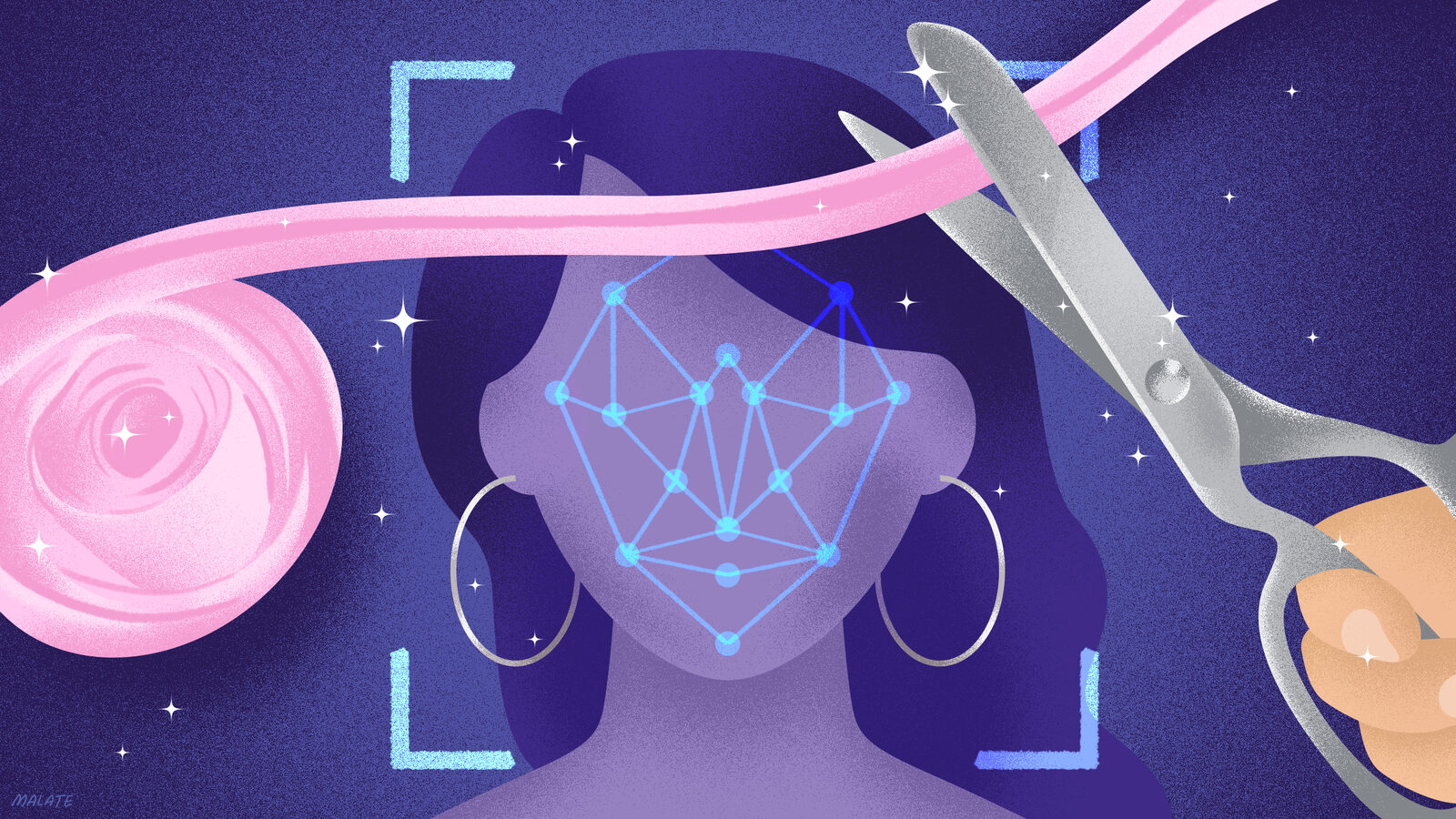
🌈 Abstract
The article discusses the ethical implications of using machine learning algorithms in physics research, particularly the issue of algorithmic bias. It highlights how biases in the training data can lead to biased outputs, and how this can have significant consequences when the technology is applied in real-world contexts. The article also explores the responsibilities of physicists in driving machine learning forward and the opportunities they have to improve the technology and its use in society.
🙋 Q&A
[01] Savannah Thais' Transformation at NeurIPS
1. What was Savannah Thais' experience at the NeurIPS conference in 2017?
- Savannah Thais attended the NeurIPS machine-learning conference in 2017, hoping to learn about techniques she could use in her doctoral work on electron identification.
- At the conference, she listened to a talk by AI researcher Kate Crawford, who discussed bias in machine-learning algorithms.
- Crawford mentioned a study showing that facial recognition technology had picked up gender and racial biases from its dataset, with women of color being 32% more likely to be misclassified than white men.
- This was a watershed moment for Thais, who had previously been introduced to machine learning through physics, but was unaware of these issues with the technology.
2. How did Thais' worldview change after the NeurIPS conference?
- After the conference, Thais pivoted to studying the ethical implications of artificial intelligence in science and society.
- She realized that algorithmic bias can influence physics results, particularly when machine-learning methods are used inappropriately.
- Thais also recognized that work done for the purpose of physics research can have broader societal implications, as the improvements in machine-learning technology will also be applied in other areas.
[02] Algorithmic Bias in Machine Learning
1. How can algorithmic bias arise in machine-learning models?
- In traditional computer models, humans specify the parameters the program needs to make decisions.
- In machine-learning algorithms, the parameters are learned from the data the algorithm is trained on.
- If the training data is biased, for example, containing more examples of white men than other demographics, the algorithm will learn to differentiate better between white men, leading to biased outputs.
2. What are the potential consequences of biased facial recognition technology?
- Facial recognition technology can be used in a variety of contexts, such as identity verification, health monitoring, and law enforcement.
- When the technology fails to work equally well for all people, it can have consequences ranging from frustration to the threat of false identification and arrest.
[03] Challenges and Opportunities for Physicists
1. What are the challenges physicists face in using machine-learning models?
- Traditional computer models have a limited number of parameters that physicists can manually tweak to get correct results.
- Machine-learning algorithms use millions of parameters that often don't correspond to real, physical characteristics, making it impossible for physicists to interpret and correct the errors.
- If physicists are not aware of these issues, they may use models for purposes beyond their capabilities, potentially undermining their research results.
2. What opportunities do physicists have in improving machine-learning technology?
- Physicists can use their technical expertise to educate citizens and policymakers on the capabilities and implications of machine-learning technology.
- Physics data is highly controlled and quantifiable, making it a perfect "sandbox" for learning to build models that avoid bias.
- Physicists can incorporate ethics into their thinking and research, helping to improve the science of machine learning itself.