
Predicting AI
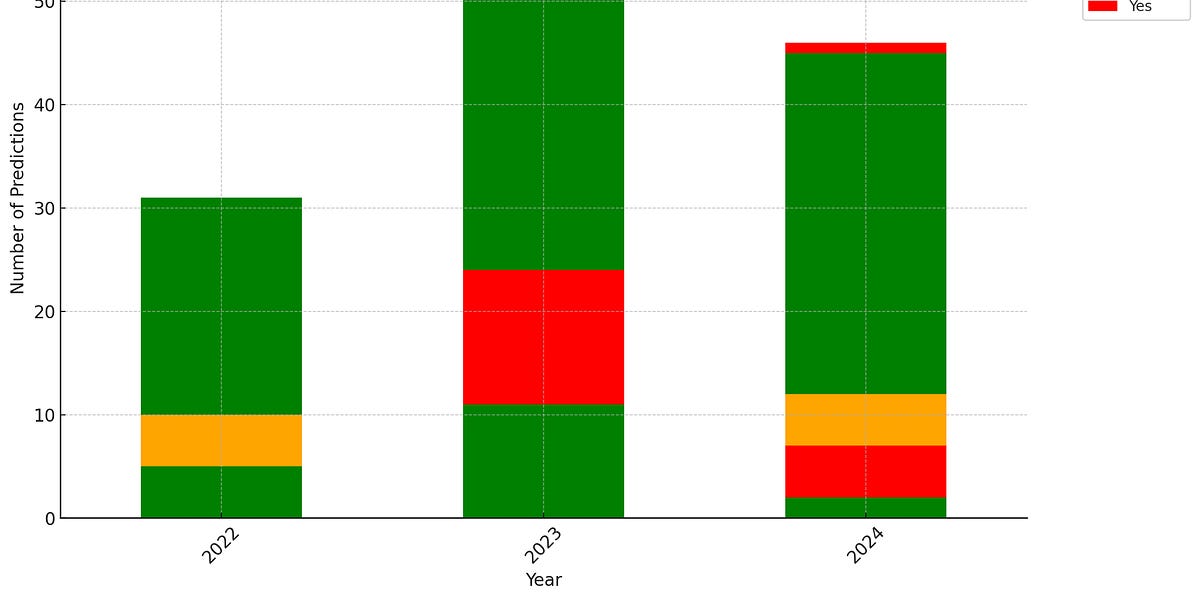
๐ Abstract
The article reflects on the author's past predictions about the development and impact of AI technology. It discusses the aspects they got right, such as the rise of open-source, multimodal models, and the importance of improving evaluation and development processes. It also covers areas where their predictions were off, like the pace of societal adjustments to AI's impact on creative industries and the job market. The author acknowledges their biases and the need to be more generous with base rates when making future predictions.
๐ Q&A
[01] The author's reflections on their past AI predictions
1. What did the author get right in their past AI predictions?
- The author got several things right, including:
- Predicting the rise of open-source, multimodal models
- Accurately forecasting technical improvements in AI capabilities
- Recognizing the iterative nature of AI development and the need to improve evaluation and development processes, especially for specific tasks
- Anticipating the rise of AI solopreneurs and the bimodal distribution of AI's impact on big tech and smaller players
- Identifying the potential of large language models (LLMs) to transform education and administrative tasks
2. What did the author get wrong in their past AI predictions?
- The author's predictions around the pace of societal adjustments to AI's impact were off, particularly in the creative industries where litigation and resistance to change has been more prevalent than expected.
- The author also thought the idea of "bullshit jobs" and automation would lead to faster changes in the job market, though the impact has not yet shown up in productivity statistics.
3. What areas did the author avoid making predictions about?
- The author stayed away from debates around AI consciousness and sentience, as well as specific technical benchmarks like when AI will be able to play Tic-Tac-Toe perfectly or win math Olympiads.
- The author also did not make many predictions about the emergence of personal assistants and new art forms enabled by AI simulations.
4. What investment thesis did the author lay out in their book, and how did it fare?
- In the book "Building God", the author outlined an investment thesis on the types of companies that would benefit from AI, including both private (e.g., Coreweave, Lambda) and public (e.g., big tech) companies.
- The author notes that the thesis on big tech being the primary beneficiary has largely played out, though the performance of some of the private companies mentioned is still "TBD".
5. What were the author's most successful and unsuccessful prediction areas?
- The author's most successful predictions were around the difficulties of scaling AI and how it would continue to transform the software industry.
- The author's less successful predictions were around the pace and nature of societal adaptations to AI, underestimating the social frictions involved in AI adoption.
[02] The author's current thoughts on the future of AI
1. What are the author's views on the future productization of AI?
- The author believes the productization of AI will continue, with systems emerging that can reason and have fewer hallucinations. The key question is whether these will be "general" AI applicable across domains or more "specific" in their capabilities.
2. What metaphor does the author use to describe large language models (LLMs)?
- The author refers to LLMs as "soft APIs", suggesting they can be built upon and integrated into various applications and workflows.
3. What are the author's main takeaways from this retrospective exercise?
- The author's main takeaway is that they underestimated the social frictions and pace of societal adaptations to AI, highlighting the need to be more generous with base rates when making future predictions.