
Artificial intelligence by mimicking natural intelligence
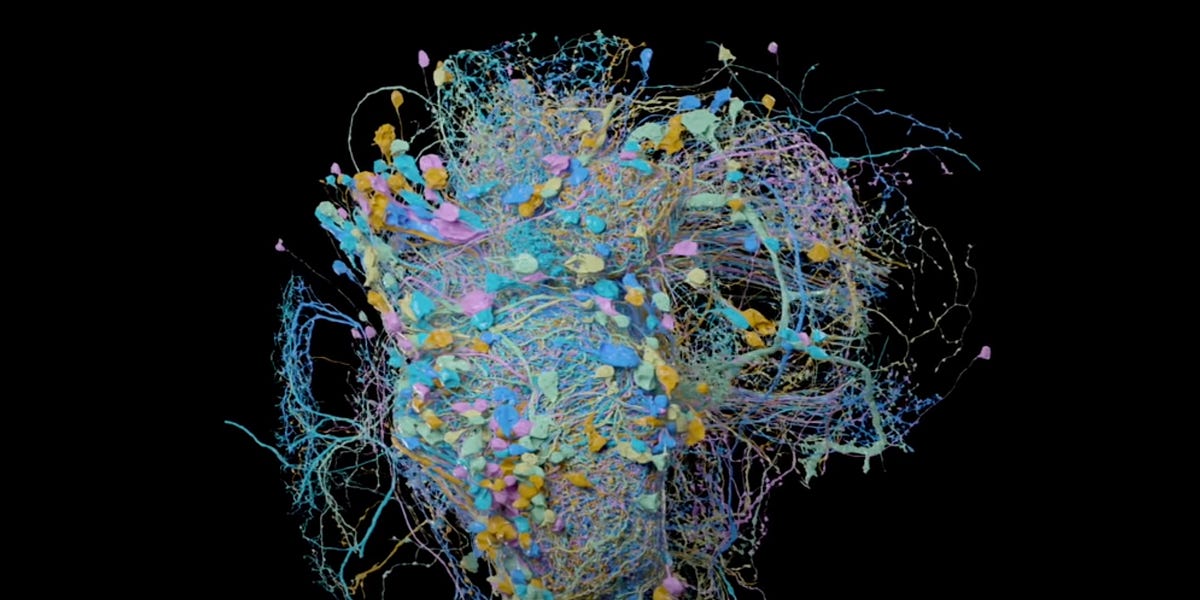
๐ Abstract
The article discusses different approaches to achieving Whole-Brain Emulation (WBE) as a path to Artificial General Intelligence (AGI), including high-fidelity (hi-fi) and low-fidelity (lo-fi) methods. It covers the key ideas, challenges, and opportunities around WBE.
๐ Q&A
[01] Hi-fi and Lo-fi Paths Toward WBE
1. What are the hi-fi and lo-fi approaches to WBE described in the article?
- Hi-fi approaches involve:
- Slicing the brain using electron microscopy, using petascale computing to label and stitch together the slices to obtain a connectome
- Simulating the connectome forward using single-neuron models of varying biological plausibility
- Simulating the body and environment to create a whole-brain-body-environment simulation
- Lo-fi approaches include:
- Top-down (or outside-in) approaches that involve:
- Measuring behavior in an animal with high accuracy
- Learning generative models of the behavior
- Embodying the behavior in a virtual agent
- Bottom-up (or inside-out) approaches that involve:
- Cloning the neural activity (electrophysiological activity of neurons) rather than the behavior
- Hybrid approaches that attempt to stitch together observed behavior and neural data
- Top-down (or outside-in) approaches that involve:
2. What are some of the challenges and open questions around hi-fi and lo-fi WBE approaches?
- For hi-fi approaches:
- Uncertainty around whether the brain is chaotic, which would make literal whole-brain emulation difficult
- Questions about how much the static connectome can capture, given factors like dendrite and synapse turnover, and non-synaptic transmission
- For lo-fi approaches:
- Challenges in scaling up bottom-up approaches like neural activity cloning to larger organisms
- Questions about the purpose and utility of WBE models at different levels of resolution or coverage
[02] Hybrid Approaches to WBE
1. What are the key ideas behind hybrid approaches to WBE?
- Hybrid approaches attempt to stitch together observed behavior and neural data, leveraging the strengths of both top-down and bottom-up approaches.
- Training neural network architectures with the right inductive biases on a combination of tasks has led to models with good one-to-one correspondence between simulated and real neural units, as seen in work on the retina and fly optic flow detection.
2. What are the potential benefits and challenges of hybrid approaches?
- Potential benefits:
- Hybrid approaches could be more feasible than pure hi-fi or lo-fi approaches in the near term.
- Combining behavioral and neural data may help overcome the limitations of each individual approach.
- Challenges:
- Identifying the right combination of tasks and inductive biases to achieve the desired level of correspondence between simulated and real neural units.
- Determining the appropriate level of resolution and coverage required for the hybrid model to be useful for neuroscience and AI applications.
Shared by Daniel Chen ยท
ยฉ 2024 NewMotor Inc.