
Backcasting From Known AI Scaling Laws
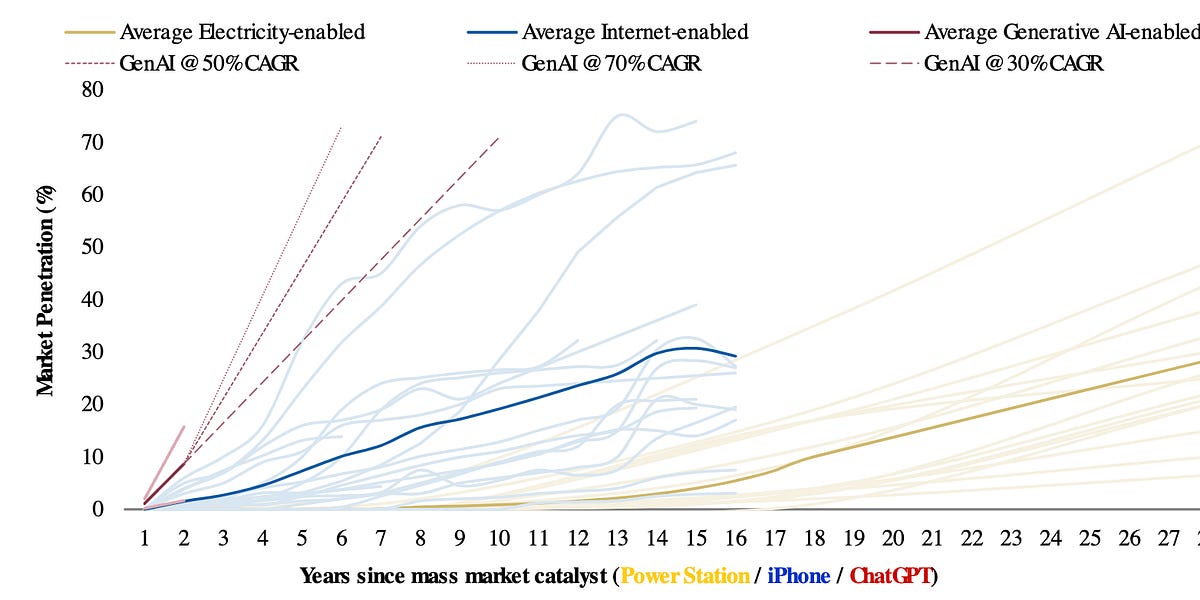
๐ Abstract
The article discusses the current state of AI scaling laws and the potential futures that entrepreneurs can realize, even without accounting for unknown unknowns. It covers the following key points:
๐ Q&A
[01] Software Synthesis and AI Scaling Laws
1. What are the key known knowns and known unknowns regarding AI scaling laws?
- We know that scaling laws are holding for now, but we don't know if they'll continue indefinitely.
- We don't know where another breakthrough in model capabilities might come from, but the most likely sources are improvements in algorithms, scaling data in novel ways, and continued construction of data centers to scale compute.
- We know we'll hit a data wall in around four years, but we don't know what new scaling techniques will be devised to compensate for the scarcity of data.
- The unknown unknowns, such as sudden advancements in capabilities that deviate from our current mapping of scaling laws, are unknowable.
2. How can entrepreneurs create value in this paradigm shift, even without accounting for unknown unknowns?
- By studying the known knowns and known unknowns, entrepreneurs can already create value in this paradigm shift.
- The framework of "backcasting" proposed by Mike Maples Jr. of Floodgate, which involves identifying inflections, backcasting from possible futures, and gathering breakthrough insights, fits well with the scaling laws and their known quantities.
[02] The "ChatGPT Moment" and Implications
1. What are the key implications of the "ChatGPT moment"?
- The "ChatGPT moment" dwarfed previous catalytic events that spurred technology diffusion, such as the adoption of internet services following the iPhone or adjacencies that electricity spawned.
- This seismic shift followed the "unhobbling" of latent model capabilities by simply building a front-end for GPT-3.5 and some instruction-tuning.
- Some argue that enhancing current frontier base model capabilities alone would deliver significant economic value, even if there's no deeper breakthrough.
2. What are the trends and implications related to the cost of inference and hardware utilization?
- The cost of inference is coming down drastically, spurred by the competitiveness of open-source models. The price reduction over 17 months corresponds to about a 79% drop in price per year.
- The physical compute required to achieve a given performance in language models is declining at a rate of 3 times per year.
- These trends have implications for the economics of AI applications, as they can be powered by the n-1 class of cheaper models as well as frontier models.
[03] Backcasting and the Role of "Seers"
1. What is the concept of backcasting, and how can it be applied to AI scaling laws?
- Backcasting is the opposite of forecasting, and it works best when you want to create something extraordinarily unique and surprising.
- Backcasting plausible, possible, and preposterous futures based on the known quantities about AI comes with the challenge of fundamentally rethinking the way we've interacted with computers for decades.
- Plausible futures are ones where agents interact with databases or digital workers replace humans for large swathes of OpEx. Possible futures are more radical in scope, leveraging technologies in groundbreaking ways. Preposterous futures are even harder to imagine.
2. What is the role of "seers" in the backcasting process?
- "Seers" are people who you believe are living in the future around a given inflection, such as entrepreneurs, researchers, academics, futurists, hobbyists, and angel investors.
- Spending time with these "seers" reinforces tech hubs and helps breathe new life into them, as seen in the case of AI and San Francisco.
- In Europe, there is a lack of "seer" density, which founders and prospective entrepreneurs find frustrating. Building communities to rectify this is an ongoing effort.
Shared by Daniel Chen ยท
ยฉ 2024 NewMotor Inc.