
The rule of four: anomalous distributions in the stoichiometries of inorganic compounds - npj Computational Materials
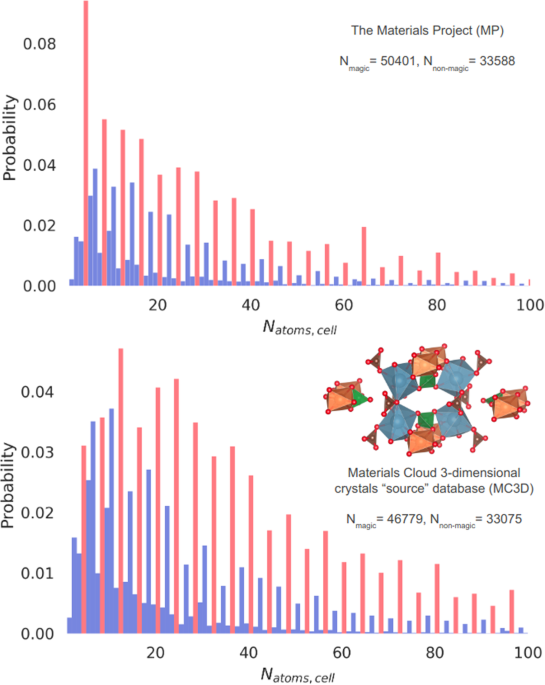
๐ Abstract
The article discusses the anomalous abundance of inorganic compounds whose primitive unit cell contains a number of atoms that is a multiple of four, a phenomenon referred to as the "rule of four" (RoF). The authors:
- Highlight the existence of the RoF, especially notable when restricting the analysis to mostly experimentally known compounds.
- Explore the relationship between the RoF and established descriptors such as formation energies, symmetries, and packing fractions.
- Conclude that the RoF does not correlate with low-energy or high-symmetry structures, but rather with low symmetries and loosely packed arrangements.
- Use symmetry-adapted machine learning techniques to accurately predict the RoF classification based on local structural symmetries, rather than global descriptors.
๐ Q&A
[01] Highlighting the Rule of Four (RoF)
1. What is the "rule of four" (RoF) that the article discusses? The RoF refers to the anomalously high relative abundance of inorganic compounds whose primitive unit cell contains a number of atoms that is a multiple of four.
2. How was the existence of the RoF observed? The RoF was observed in two different databases of inorganic crystal structures: the Materials Project (MP) database and the Materials Cloud 3-dimensional crystal structures 'source' database (MC3D-source).
3. What percentage of structures in the databases follow the RoF? As shown in Table 1, around 59% of structures in the MP database and 57% in the MC3D-source database have a primitive unit cell with a multiple of 4 atoms.
[02] Exploring Relationships with Structural Descriptors
1. Did the RoF correlate with formation energy of the compounds? No, the analysis did not find any correlation between the RoF and the formation energy of the compounds.
2. How did the RoF relate to the symmetry of the crystal structures? The RoF structures were found to have lower symmetries, being mostly grouped in the lowest symmetry point groups, compared to the non-RoF structures which had a higher abundance in the high symmetry point groups.
3. What other structural descriptors were analyzed in relation to the RoF? Other descriptors analyzed include:
- Number of atomic species in the compound
- Ratio of smallest to largest atomic radii
- Packing fraction of the structures
These analyses suggested that RoF structures tend to have greater variance in atomic sizes and lower packing fractions compared to non-RoF structures.
[03] Symmetry-Adapted Machine Learning Analysis
1. What machine learning techniques were used to further analyze the RoF? The authors used Smooth Overlap of Atomic Positions (SOAP) vectors to represent the local atomic environments, and then employed Principal Covariates Regression (PCovR) and Random Forest classification to study the relationship between the RoF and local structural symmetries.
2. What did the PCovR analysis reveal about the energetics of RoF vs non-RoF structures? The PCovR analysis showed that for structurally similar compounds, there was no significant difference in formation energy between RoF and non-RoF samples.
3. How accurately could the RoF be predicted using only local structural descriptors? The Random Forest classifier was able to predict the RoF classification with an accuracy of 87% using only information about the local structural symmetries, without requiring global structural descriptors.
[04] Conclusions
1. What were the key findings regarding the origins of the RoF? The authors were unable to find a fully satisfactory explanation for the anomalous distribution of the RoF. While they explored many avenues, including energetics, symmetries, and packing, the RoF could not be conclusively correlated with any of the traditional materials science descriptors analyzed.
2. What was the significance of the study? The study provides a valuable reference for further systematic studies targeting the classification of materials' features using machine learning approaches. It also serves as a starting point for future investigations into understanding the origins of the RoF phenomenon.