
The Shifting Dynamics & Meta-Moats of AI - Michael Dempsey: Blog
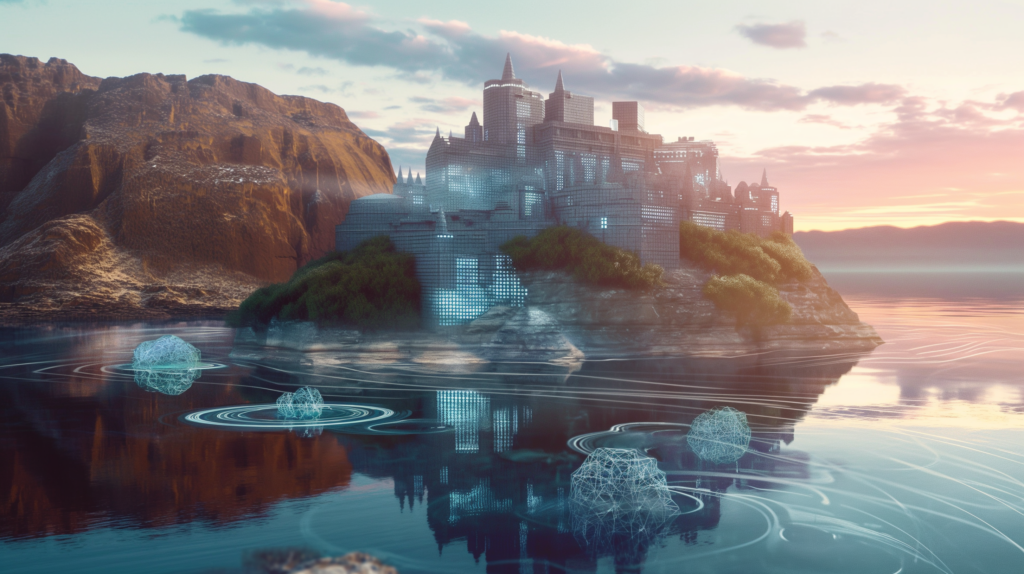
๐ Abstract
The article discusses the key considerations and strategies for building successful AI companies in the current landscape. It covers topics such as the importance of speed, data flywheels, synthetic data, talent dynamics, and the need for a long-term, multi-faceted approach to building sustainable moats.
๐ Q&A
[01] The Turing Transformation
1. What are the key learnings the author has gained about company and moat building in AI in 2024 and onward?
- The author has gained the following key learnings:
- AI technical approaches and the industry as a whole have become increasingly consensus-driven, with Transformers leading the way and various iterations getting quickly coalesced around.
- Every AI company will face material competition in the next few years, either directly or through "death by a thousand cuts". Startups need to continue standing out amidst this noise.
- Building an AI company requires doing all the things great software companies do, but faster and at a very high level, potentially implementing or creating things that have never been done before.
- The best AI companies will need to be both elite sprinters and the fastest marathon runners, maintaining velocity over multiple years.
2. How does the author characterize the speed and complexity of building AI companies?
- The author states that in reality, AI companies are perhaps some of the most complex businesses being built in tech currently. Doing core AI model R&D requires playing "4D Chess" around research communities, capital, talent, competition, and commercialization.
- Founders have to execute at a rate that outpaces natural industry-wide compression and erosion of advantages, often leading to many companies competing on the same, eventually inconsequential axis of competition.
3. What are the potential fail states the author identifies for AI companies taking a short-term approach?
- Building products that create only incremental value on top of short-term base model performance, instead of leveraging increases in base model performance to materially increase product value.
- Building products with a "x but with AI" skeuomorphic framing, instead of building novel things that are "y" because of AI.
- Getting distracted fighting the wrong battles and taking advantage of false signs of early durable product-market fit, only to get leapfrogged or destroyed by other startups or companies playing long-term games.
[02] Data Flywheels
1. What has the author learned about the value of data and scale in AI?
- Pairing multimodality and scaling has taught that a large amount of ROI from AI can come from "connecting the dots" of existing data today. Pre-training a large model and then fine-tuning it may not be the short to mid-term moat that was once believed.
- LLMs have not materially shown intelligence outside of training data distribution, and across non-language-only tasks, data and scale are not nearly maximized to drive performance.
2. What types of companies does the author find increasingly attractive as AI investments?
- Companies that aim to own more of the stack than originally anticipated, including the entire stack and building an AI-enabled operating company.
- Companies in industries where data is structurally locked within incumbents with little computational excellence (e.g., healthcare, bio, industrials), if they can navigate partnerships and build custom workflows to generate novel datasets.
3. How does the author view the role of synthetic data in AI companies?
- The author believes synthetic data will enable companies to improve their models more efficiently than gathering varied data quality and deploying compute.
- For companies operating in sparse data environments, synthetic data will enable them to reach the point of deployment faster or make better "collect vs. buy vs. generate" data decisions.
- The author hypothesizes that unfortunately, synthetic data won't be prioritized enough in the near-term, and by the time it is, it may be too late.
[03] Market Timing & Talent
1. What shifts in the ideal team compositions within AI startups has the author observed?
- AI has started to pull talent from all over, allowing very talented engineers to figure out how to problem-solve with the constraints brought on by building in AI. These constraints are more familiar to engineers, especially those who have worked at startups, than researchers and engineers from larger megatech labs.
2. How does the author characterize the current state of talent dynamics in the AI industry?
- There is a lack of consensus amongst talent around what companies that own parts of the AI stack are unassailable versus not, leading to a bifurcation of talent that believes they must work at either a Frontier lab or an unexplored category, versus others who think the next wave of innovation will come from what gets built on top of the models.
- The author sees a dynamic where many will spin out of the companies that caused the Cambrian Explosions in AI to re-invent the wheel marginally better than their former employers/scaling incumbents, which the author views as a disastrous way to lose money.
[04] The Fallacy of Soon To Be Solved & The Value of Contrarian Approaches
1. What is the author's perspective on the industry's tendency to extrapolate progress to its end state, and how does this relate to potential "final mover advantages" in AI?
- The author believes the technology industry has gotten very good at extrapolating progress to its end state, but is still sub-par at projecting time horizons and the "random" walks that might get us to these end states.
- The author thinks there could be some large changes lurking that are unknown, and there could be final mover advantages that are not yet appreciated within AI.
2. How does the author view the consolidation of talent in deep tech, and how does this relate to the bifurcation of views on the future of AI?
- The author believes that within deep tech, talent seems to consolidate at a far faster rate than in other areas of technology. As AI continues to progress and the nature of how people think about the space bifurcates, talent advantages will begin to materially accrue to those that can make credible cases for their camp of thought.
3. How does the author summarize the "Rorschach Test" of someone's conviction in their view of the future of AI?
- The author states that whether someone intensely covets a job at OpenAI and nowhere else is perhaps a great Rorschach Test for someone's conviction in their view of the future of AI.