
Correlations and Coin Flips
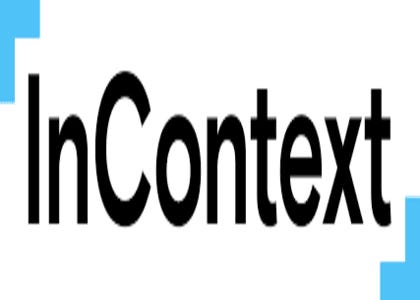
🌈 Abstract
The article discusses the limitations of using "variance explained" (r²) as a metric for understanding the practical significance of relationships between variables. It introduces alternative metrics like the binomial effect size display (BESD) proposed by Rosenthal and Rubin, and explores the challenges in using the BESD to intuitively convey the real-world impact of small effects. The article emphasizes the importance of considering the appropriate context when interpreting effect sizes, as a "small" correlation can sometimes have large practical implications.
🙋 Q&A
[01] Correlations and Coin Flips
1. What are the key issues with using "variance explained" (r²) as a metric?
- "Variance explained" (r²) is a misleading metric because its scale is unintuitive - a correlation denotes the gain in Y if you change X by one standard deviation, but squaring this value can lead to severe underestimates of the practical and theoretical significance of the relationship.
- The article uses the analogy of a friend giving you a ride for the first 5 miles of a 10-mile trip - you're only a quarter of the way there, not halfway, even though the r² would be 0.25.
2. What is the binomial effect size display (BESD) and how does it aim to address the issues with r²?
- The BESD is an alternative metric proposed by Rosenthal and Rubin to convey how something that explains a small share of the variance can have large practical implications.
- The BESD shows the increase in success rate (e.g. from 34% to 66%) associated with a given correlation, to illustrate the real-world impact.
- Rosenthal provided examples of how the BESD can demonstrate the practical significance of small correlations in various medical contexts.
3. What are the limitations of the BESD approach?
- The article demonstrates through simulations that the BESD-implied correlation does not always accurately reflect the true underlying correlation, especially as the true correlation increases.
- The BESD assumes uniform marginal distributions, which may not always hold in real-world data.
- The article concludes that there is no single, universally applicable statistic that can perfectly translate an effect size into an intuitive real-world impact.
[02] Effects in Context
1. How can a "small to modest" correlation explain a major difference in trait means between two groups?
- The article uses the example of Pygmy height to illustrate how a relatively small correlation (e.g. 0.34) between admixture from a taller group and height can account for a large mean height difference between Pygmies and their neighbors.
- This is because the standard deviation of the admixture variable within the Pygmy group is much smaller than the mean difference in height between Pygmies and the taller neighboring group.
2. How can a "small" correlation between social media use and depression lead to a large increase in depression rates?
- The article explains that in a liability threshold model, a small correlation (e.g. 0.16) between social media use and depression can result in a large increase in the overall depression rate when social media is introduced, compared to a world without social media.
3. What is the key principle the article suggests for communicating scientific results with "small" effect sizes?
- The article suggests that whenever possible, researchers should place effects into an appropriate context before presenting them in terms of well-known, frequently misunderstood effect size metrics like r or r².
- This helps avoid the misconception that "small" effects are always insignificant, and allows the real-world practical implications to be better understood.