
Why data-driven product decisions are hard (sometimes impossible)
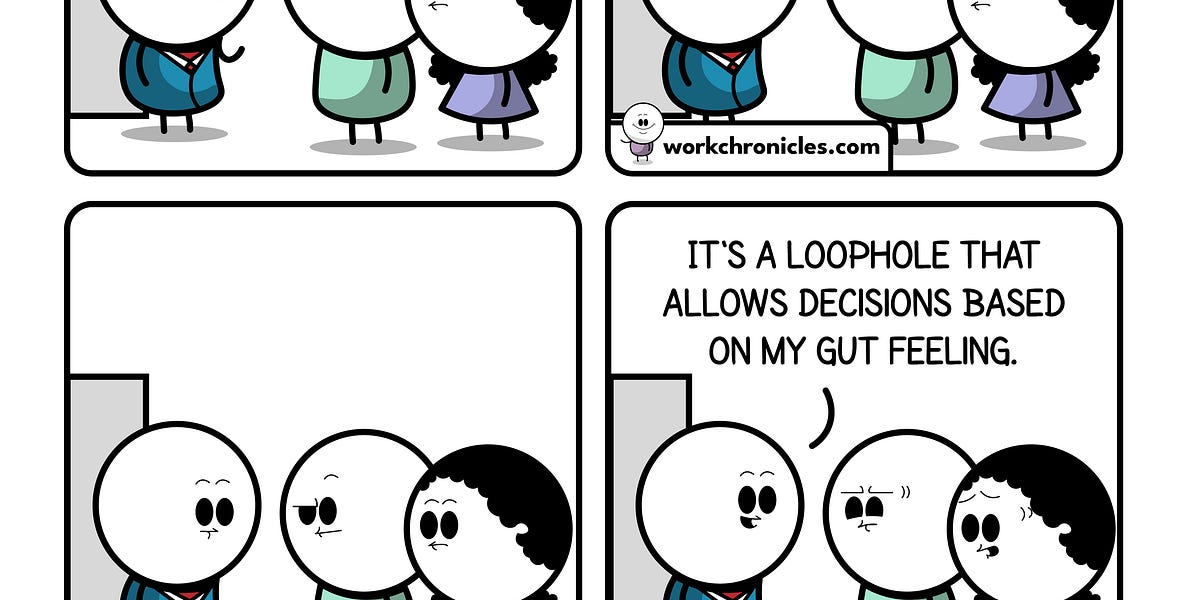
๐ Abstract
The article discusses the challenges and limitations of being data-driven in product decision-making. It explores the paradox of using data to make decisions and the various problems that can arise, such as measuring the wrong metrics, analysis paralysis, the tyranny of the majority, and the difficulty in establishing causation. The article also argues that in certain situations, particularly for startups competing with larger tech companies, relying more on intuition and making bold moves may be a better strategy than being strictly data-driven.
๐ Q&A
[01] The problem with being data-driven
1. What are some of the common problems encountered when trying to be data-driven in product decision-making?
- Measuring yesterday's data when you should be thinking about tomorrow's market
- Analysis paralysis due to the cost and time required to acquire and analyze data
- Intuition being faster than A/B tests
- The tyranny of the majority when aggregating user data into segments
- A/B tests being good at measuring big things quickly but not other important metrics
- Noise in the data from factors like seasonality, competitor launches, etc.
- Historical data not always predicting future trends, especially with emerging technologies
- Mistaking correlation for causation when analyzing user behavior
2. Why is truth-seeking so difficult when there is so much data available about how people use products? The article suggests that the "fog of war" when making product decisions leads to a lot of excuses and speculation rather than clear insights from the data. This is due to the various limitations and problems with being data-driven.
3. What are the root causes for the problems encountered when trying to be data-driven? The root causes include:
- Measuring the wrong metrics that don't reflect the future market
- The cost and time required for in-depth data analysis leading to "analysis paralysis"
- The speed of intuition being faster than the slow pace of A/B testing
- Aggregating user data into segments that may not accurately represent important minority segments
- A/B tests being good at measuring big things quickly but not nuanced metrics
- Noise in the data from external factors
- Historical data not always predicting future trends, especially with emerging technologies
- Mistaking correlation for causation when analyzing user behavior
[02] Competing against tech's dominant culture
1. What is the dominant culture in the tech industry when it comes to product management? The dominant culture is one of data, optimization, and taking small concrete steps to move easily measurable metrics. This has been driven by the success of product management cultures at companies like Google and Meta.
2. How can a startup compete against this dominant data-driven culture? The article suggests that startups competing against larger tech companies cannot simply race them in the same data-driven way. Instead, startups may need to:
- Rely more on intuition rather than waiting for data
- Make super fast decisions rather than incremental ones
- Pursue big moves rather than small optimizations
3. What are the potential benefits of a more intuition-driven approach for startups? The article argues that in certain zero-to-one situations, particularly for startups competing with larger tech companies, being "data-ignorant" and relying more on intuition and bold moves may be a better strategy than being strictly data-driven. This can allow startups to move faster and make more transformative changes than the incremental optimizations of their larger competitors.