
Looking for AI use-cases — Benedict Evans
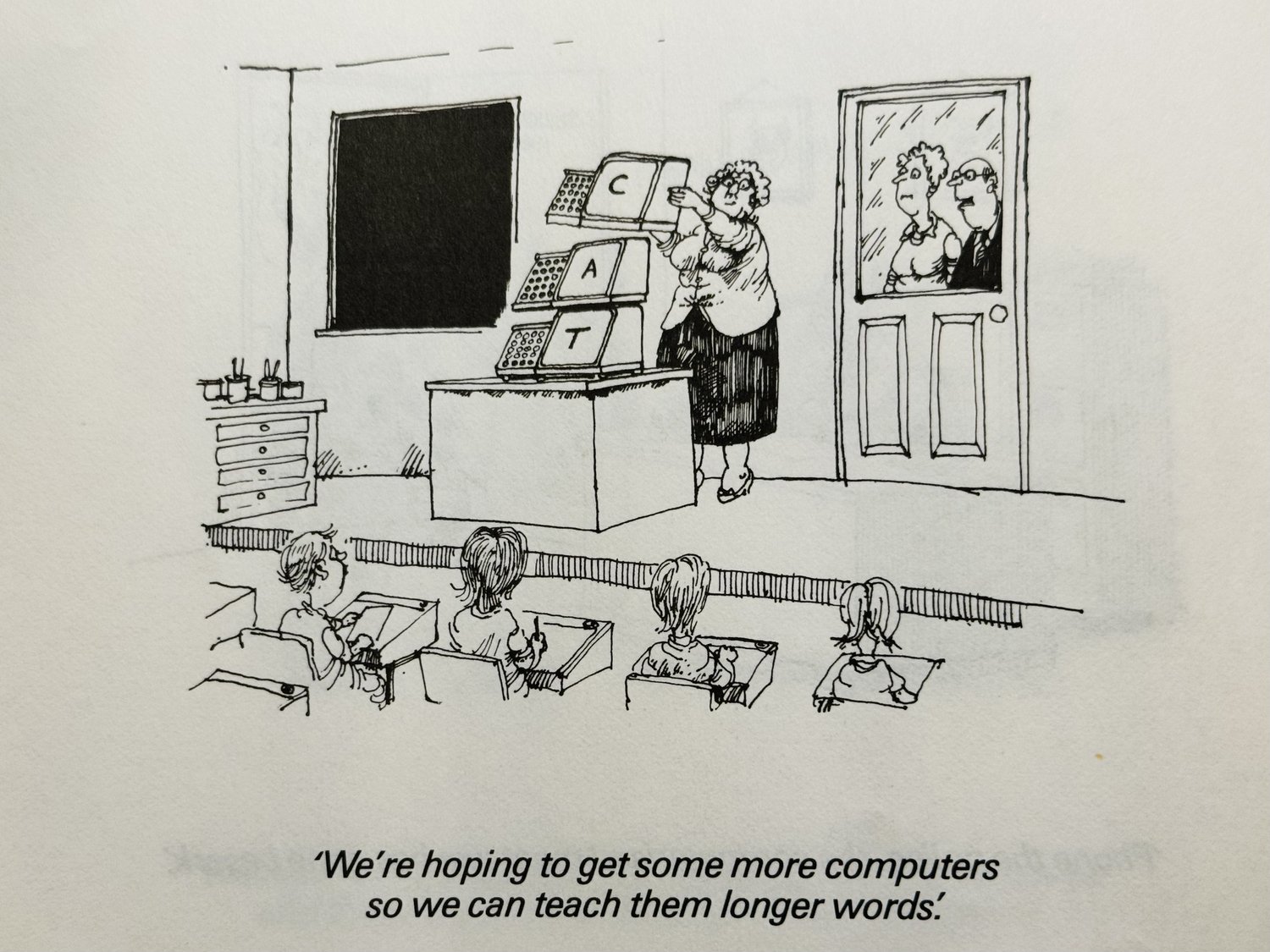
🌈 Abstract
The article discusses the challenges of finding practical use cases for new technologies, particularly in the context of the recent advancements in generative AI models like ChatGPT. It explores the parallels between the adoption of spreadsheets, word processors, and other productivity tools, and the current landscape of AI-powered applications.
🙋 Q&A
[01] Looking for AI use-cases
1. What are the key points made about the adoption of new technologies like ChatGPT?
- The author has not yet found a use case for ChatGPT and other chatbots that matches their own needs, and suggests they are not alone in this experience based on survey data.
- The author notes that a lot of people have tried these new technologies, but the question is how much they are actually used and for what purposes.
- The author suggests that the transformative potential of these models is in their ability to handle a wide range of tasks without the need to write specific software for each one, but that this potential has not yet been fully realized.
2. What are the two main problems the author identifies with the thesis of these models being able to handle "any" use case?
- The narrow problem is that the models are not yet good enough, with issues like getting stuck, making errors, and producing "hallucinations" that are not always reliable.
- The deeper problem is that users need to be able to imagine and identify the use cases that could be automated, which is not always easy and requires a shift in mindset and workflow.
3. What parallels does the author draw between the adoption of new technologies like ChatGPT and previous productivity tools like spreadsheets?
- The author notes that it took time for people to realize the full potential of tools like Google and spreadsheets, as users initially tried to fit them into their existing workflows before gradually adapting their workflows to take advantage of the new capabilities.
- The author suggests that, like with previous productivity tools, it is not the user's job to figure out how to use a new technology, but rather the responsibility of the technology providers to identify and demonstrate the use cases.
[02] The challenges of realizing the potential of generative AI
1. What are the key points made about the cognitive dissonance between the claims of generative AI's potential and the current reality?
- The author notes that while OpenAI and Anthropic claim we are close to general-purpose autonomous agents, in reality there is a "Cambrian Explosion" of startups using their APIs to build single-purpose dedicated apps, much like previous generations did with SQL.
- The author suggests that generative AI may be more akin to providing "another set of interns" that can recognize and create content, but that we still need to work out what specific use cases to apply them to.
2. What analogy does the author use to illustrate the challenges of automating complex, multi-step tasks?
- The author uses the example of trying to automate the task of finding a specific time-series dataset on US employment by occupation, noting the embodied knowledge and iterative problem-solving required to navigate the complexities of data sources like the US Census.
3. What are the potential solutions the author suggests for bridging the gap between the potential of generative AI and the need for specific use cases?
- The author suggests that the solution may lie in capturing the embodied knowledge and workflow in dedicated apps or services, where the choices and options are pre-defined by someone who understands the problem domain.
- Alternatively, the author proposes the idea of "generative GUIs" that could dynamically adapt to the user's needs, or the need for a new generation of "Dan Bricklins" to identify and build applications around specific use cases.