
Why AIs Need to Stop and Think Before They Answer
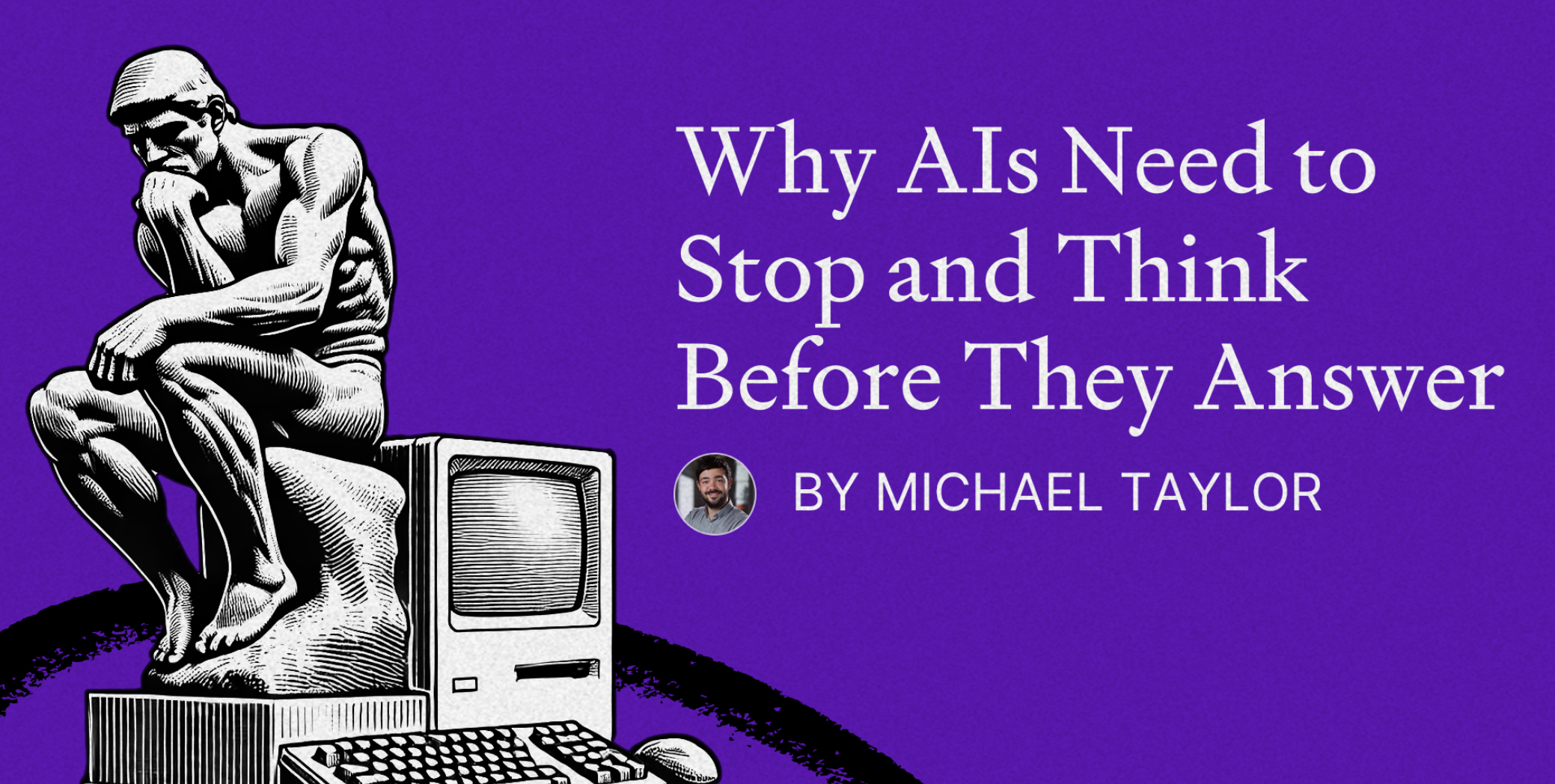
๐ Abstract
The article discusses the concept of "chain of thought prompting" - a method for getting the most out of AI assistants like ChatGPT. It explores how this technique can lead to more sophisticated and accurate results compared to simply asking the AI to perform a task without any planning or reasoning steps.
๐ Q&A
[01] Chain of Thought Prompting
1. What is chain of thought prompting, and how does it work?
- Chain of thought prompting is a method that encourages AI models to engage in a step-by-step reasoning process, similar to how humans approach complex problems.
- It involves prompting the AI to "think through" a problem or task, rather than just providing a direct answer. This allows the AI to plan, research, and apply logic before generating a response.
- Implementing chain of thought can lead to significantly better results compared to standard prompting, as it taps into the AI's ability to engage in more deliberate, "System 2" thinking.
2. Why is chain of thought effective for improving AI performance?
- By default, AI models tend to respond in an instant, using patterns in their training data, which can lead to inaccurate or incomplete results.
- Applying chain of thought prompting encourages the AI to slow down and go through a more thoughtful, step-by-step process, similar to how humans approach complex problems.
- This allows the AI to better understand the problem, make reasonable assumptions, and apply logic to arrive at a more sophisticated and accurate response.
3. How can users implement chain of thought prompting?
- Users can implement chain of thought by appending prompts like "Let's think step by step" or providing examples of the reasoning steps they want the AI to take.
- This signals to the AI model that it should engage in a more deliberate thinking process before generating a response.
- The article provides examples of how chain of thought prompting can be used to improve the performance of AI models on tasks like counting letters in a word or solving Fermi problems.
[02] Fermi Problems and Reasoning
1. What are Fermi problems, and how do they relate to chain of thought prompting?
- Fermi problems are open-ended estimation problems that require breaking down a complex problem into smaller, more manageable parts and making educated guesses about key variables.
- The article explains that Fermi problems are similar to the chain of thought approach, as they involve a step-by-step reasoning process to arrive at a reasonable approximation of the answer.
- The article uses the example of estimating the number of piano tuners in New York City to demonstrate how chain of thought prompting can be applied to solve Fermi problems with AI models.
2. How do Fermi problems and chain of thought prompting relate to human decision-making?
- The article draws a parallel between Fermi problems and the "System 1" and "System 2" thinking processes described in Daniel Kahneman's book "Thinking, Fast and Slow".
- System 1 thinking is the fast, intuitive decision-making that humans often use for everyday tasks, while System 2 thinking is the slower, more deliberate process used for complex problem-solving.
- The article suggests that by implementing chain of thought prompting, AI models can better emulate the System 2 thinking process, leading to more sophisticated and accurate results.
3. What are the potential benefits and limitations of using chain of thought prompting with AI models?
- The article notes that while chain of thought prompting can lead to significantly better results, it also comes with increased costs in terms of token usage and completion time.
- However, the article suggests that as AI models continue to scale and become more affordable, the benefits of chain of thought prompting may outweigh the costs, allowing for more advanced reasoning and problem-solving capabilities.
- The article also mentions emerging techniques like Quiet-STaR that may further improve the reasoning abilities of large language models, unlocking even more advanced use cases.