
Researchers leverage shadows to model 3D scenes, including objects blocked from view
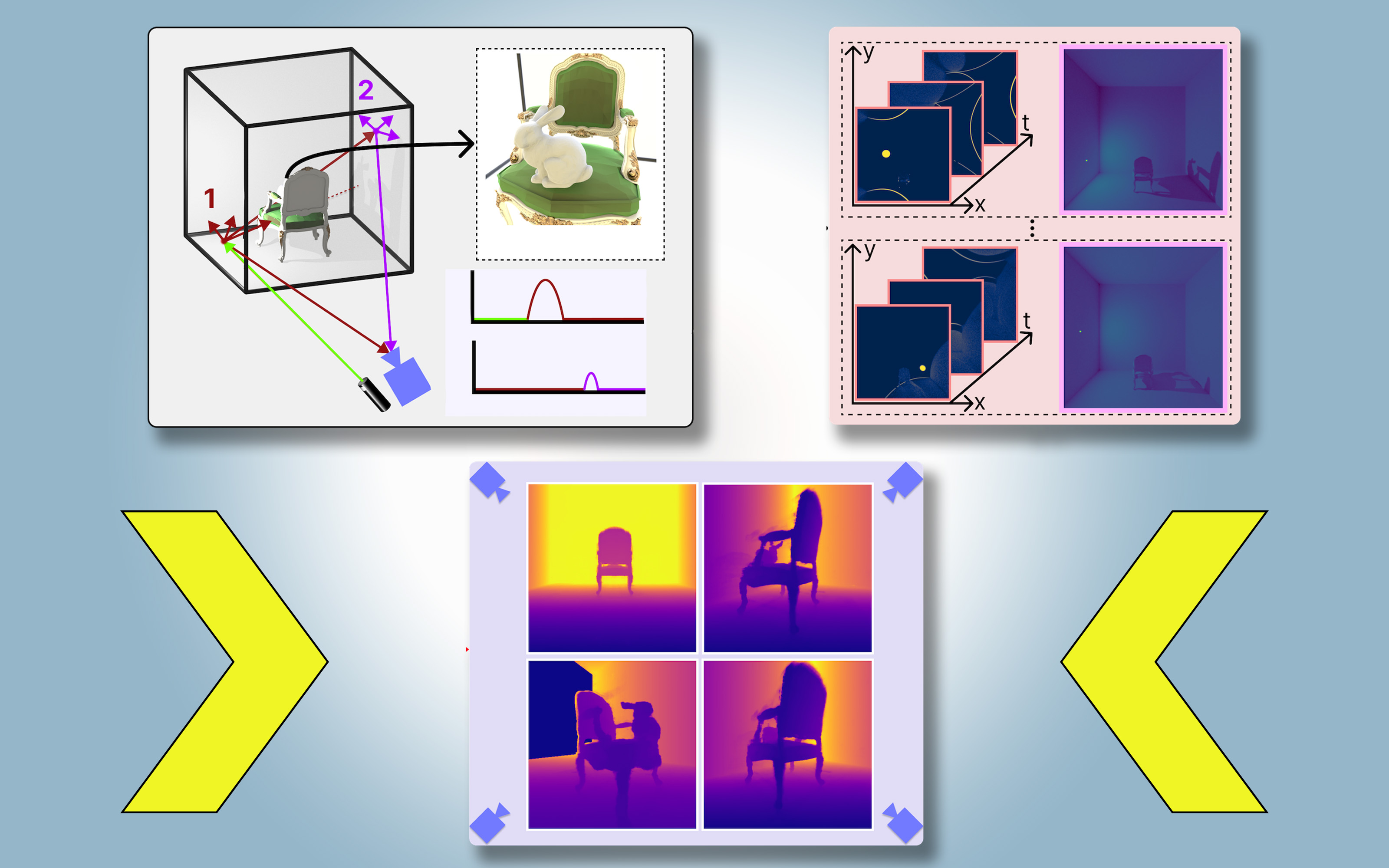
๐ Abstract
The article discusses a computer vision technique called PlatoNeRF, developed by researchers from MIT and Meta, that can create physically accurate 3D models of an entire scene, including areas blocked from view, using images from a single camera position. The technique uses shadows and multibounce lidar technology combined with machine learning to reconstruct 3D geometry more accurately than existing AI techniques.
๐ Q&A
[01] Reconstructing 3D Scenes from Single Camera Viewpoint
1. What are the key challenges in reconstructing a full 3D scene from a single camera viewpoint?
- Some machine learning approaches use generative AI models that can hallucinate objects that aren't really there in the occluded regions.
- Other approaches try to infer the shapes of hidden objects using shadows in a color image, but these methods can struggle when shadows are hard to see.
2. How does PlatoNeRF address these challenges?
- PlatoNeRF uses a single-photon lidar to capture information about the scene, including depth and shadows, by tracing the secondary rays of light that bounce off the target point to other points in the scene.
- By calculating how long it takes light to bounce twice and return to the lidar sensor, PlatoNeRF can infer the geometry of hidden objects based on the location of the shadows.
3. What are the key advantages of PlatoNeRF compared to other methods?
- PlatoNeRF outperforms techniques that only use lidar or only use a NeRF with a color image, especially when the lidar sensor has lower resolution.
- The combination of multibounce lidar and a neural radiance field (NeRF) model allows for highly accurate scene reconstructions through the ability to interpolate novel views of the scene.
[02] Applications of PlatoNeRF
1. What are some potential applications of the PlatoNeRF technology?
- Improving the safety of autonomous vehicles by enabling them to see around the car ahead and apply brakes sooner in case of an accident.
- Making AR/VR headsets more efficient by enabling users to model the geometry of a room without the need to walk around taking measurements.
- Helping warehouse robots find items in cluttered environments faster.
2. How could PlatoNeRF be further improved in the future?
- The researchers want to try tracking more than two bounces of light to see how that could improve scene reconstructions.
- They are interested in applying more deep learning techniques and combining PlatoNeRF with color image measurements to capture texture information.