
How To Price A Data Asset
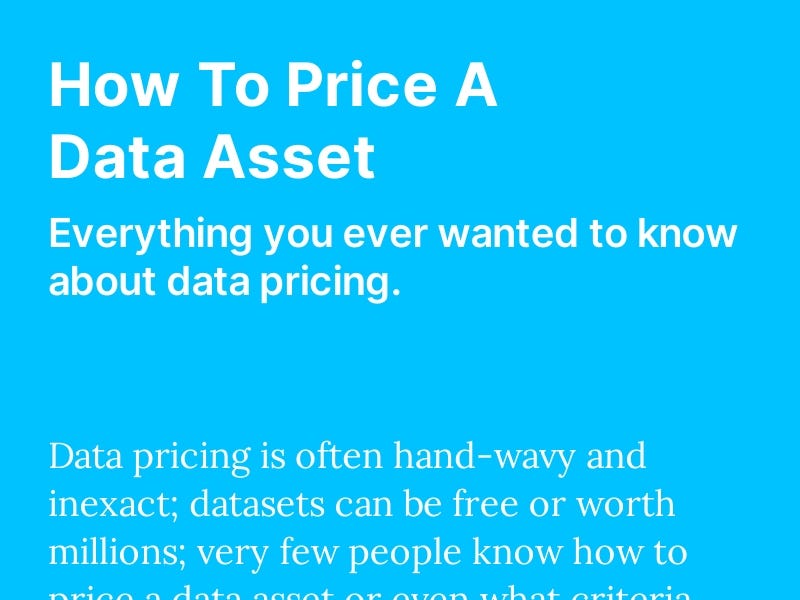
๐ Abstract
The article discusses the challenges and principles of pricing data assets, drawing on the author's experience as the co-founder and chief data officer of a data marketplace. It covers key axioms of data value, implications for data pricing, and the impact of AI on data economics.
๐ Q&A
[01] Axioms of Data Value
1. What are the key axioms of data value discussed in the article?
- Data has no innate value - its value comes from what can be done with it
- Data value depends on the use case and the user
- Data is fundamentally additive, unlike software
- Data is rivalrous, not non-rival, as commonly believed
- Data assets have well-defined lifecycles from early adoption to becoming table stakes
- The marginal lift that data provides is what determines its value
2. How does the author explain the concept of data being rivalrous? The author argues that data is not truly non-rival, as commonly believed. Even though data can be easily duplicated, the value of data lies in what can be done with it. If a dataset holds unpriced information content, only one party can act on it profitably before the opportunity goes away. Data owners often try to keep valuable datasets exclusive and protected, which wouldn't happen if data were truly non-rival.
3. What are the different stages of the data asset lifecycle described in the article?
- Early stage: Dataset and market are immature, with little value and few transactions.
- Early adopter stage: Dataset provides an "alpha" or edge, but to a narrow audience.
- Decay stage: Substitutes proliferate, and the dataset's alpha advantage decays.
- Table stakes stage: Dataset becomes essential, with widespread usage and higher prices.
[02] Implications for Data Pricing
1. Why is unique/proprietary data so valuable according to the article? Unique data is universally additive, can be combined with existing datasets to increase utility, and the data owner can control its lifecycle to maximize value. If a unique dataset becomes table stakes, the owner has monopoly power to collect a "tax" on an entire industry.
2. How does the author explain the concept of "functional substitutes" for data? Functional substitutes are completely different datasets that offer similar insights or value. For example, foot traffic data, email receipts, and credit card transactions can all provide insights into consumer purchasing behavior. These functionally substitute datasets compete with each other, even though they are very different in nature.
3. Why do traditional software pricing models often fail for data products? Traditional models like pricing by seat, feature, or raw volume don't work well for data, as data value doesn't scale linearly with these factors. The author suggests alternative approaches like pricing by structured volume, quality, access, use case, customer scale, or business unit.
4. How can wrapping data in software or services help with data pricing? Wrapping data into a software application or service allows the data owner to better link the data's value to the value delivered, and enables the use of traditional software pricing models like per-seat or per-action. This can make data pricing more effective.
5. How does the author describe the importance of data quality for pricing? Data quality is multi-dimensional, with different attributes like accuracy, coverage, structure, and annotations being more important for different use cases (e.g. finance, advertising, AI). Data owners can price-discriminate based on these quality factors, and can also improve quality to boost data value.
[03] Impact of AI on Data Economics
1. How does the author describe the importance of data quantity for AI use cases? For AI training, quantity of data often matters more than quality. There seems to be no upper limit to how much better models become with more training data, even if the additional data is "good enough" rather than high-quality. This shifts the balance towards data quantity over quality for AI applications.
2. How does the author suggest data owners can address the challenge of one-time AI training data usage? The author suggests that building a "data flywheel" - a perpetual data machine that generates or captures a steady stream of new data - can help create recurring revenue opportunities. Synthetic data pipelines are also seen as a way to generate unlimited high-quality training data at lower costs.
3. How does the author explain the concept of "legibility" and its impact on data market size? The more legible a dataset is, meaning the easier it is to objectively compute its ROI, the larger its potential market. Legible datasets tend to have more customers, higher prices, and lower acquisition costs. The author suggests AI training data may need to reach a similar level of legibility as finance and advertising data to unlock large markets.