
Testing theory of mind in large language models and humans - Nature Human Behaviour
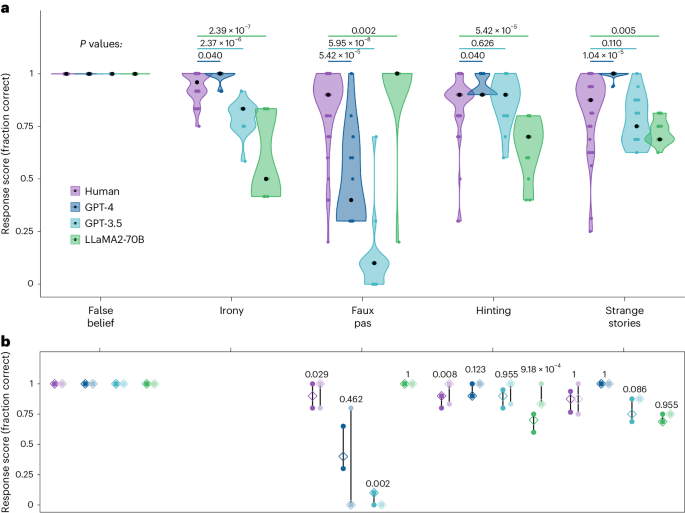
๐ Abstract
The article examines the performance of large language models (LLMs) such as GPT-4, GPT-3.5, and LLaMA2-Chat on a comprehensive battery of theory of mind tests and compares their results to a large sample of human participants. The study finds that while GPT-4 and GPT-3.5 exhibit impressive performance on many theory of mind tasks, they struggle with the faux pas test, which requires understanding the speaker's false belief. Further experiments reveal that this failure is not due to an inability to infer the speaker's mental state, but rather an overly conservative response style that prevents the models from committing to the most likely explanation. The article discusses the implications of these findings for understanding the nature of social reasoning in LLMs and highlights the importance of using systematic experimental approaches to study machine behavior.
๐ Q&A
[01] People's Theory of Mind
1. What is theory of mind and why is it important for human social interactions?
- Theory of mind refers to the ability to track and reason about other people's mental states, such as their beliefs, desires, and intentions.
- It is central to human social interactions, enabling us to communicate, empathize, and make social decisions.
2. What are some of the key tasks used to study theory of mind in humans?
- The article mentions several tasks, including:
- False belief tasks, which test the ability to infer a character's belief that differs from reality
- Irony comprehension tasks, which test the ability to understand the speaker's intended, non-literal meaning
- Faux pas tasks, which test the ability to recognize when a speaker has said something inappropriate without realizing it
- Hinting tasks, which test the ability to understand indirect speech acts
- Strange stories, which test more advanced mentalizing abilities like reasoning about misdirection, manipulation, and higher-order mental states.
3. How have recent advances in large language models (LLMs) like GPT-4 and GPT-3.5 impacted the study of artificial theory of mind?
- LLMs have shown promising performance on many theory of mind tasks, suggesting the possibility of artificial theory of mind.
- However, the mixed success of these models, along with their vulnerability to small perturbations, has raised concerns about the robustness and interpretability of their social reasoning abilities.
[02] Experimental Approach and Findings
1. What was the key goal and approach of the study?
- The study aimed to systematically investigate the capacities and limits of LLMs on a wide range of theory of mind tasks, using a diverse set of measures and comparing performance to a large sample of human participants.
2. What were the key findings regarding the performance of the LLMs compared to humans?
- GPT-4 and GPT-3.5 exhibited impressive performance on many theory of mind tasks, exceeding human levels on some tests (irony, hinting, strange stories).
- However, both GPT models struggled on the faux pas test, which requires understanding the speaker's false belief.
- In contrast, the LLaMA2-70B model outperformed humans on the faux pas test, but performed poorly on other tasks.
3. What did the follow-up experiments reveal about the nature of the GPT models' failures on the faux pas test?
- The follow-up experiments suggested that the GPT models' failures were not due to an inability to infer the speaker's mental state, but rather an overly conservative response style that prevented them from committing to the most likely explanation.
4. What are the three hypotheses proposed to explain the GPT models' performance on the faux pas test?
- The failure of inference hypothesis: the models fail to generate inferences about the speaker's mental state.
- The Buridan's ass hypothesis: the models can infer the mental states but cannot choose between them.
- The hyperconservatism hypothesis: the models can infer the mental states but refrain from committing to a single explanation due to an excess of caution.
[03] Implications and Future Directions
1. What are the key implications of the findings for understanding the nature of social reasoning in LLMs?
- The findings highlight a dissociation between competence (the technical sophistication to compute mentalistic-like inferences) and performance (the tendency to respond conservatively and not commit to the most likely explanation).
- This suggests fundamental differences in how humans and LLMs trade off the costs of social uncertainty against the costs of prolonged deliberation.
2. What are the potential implications for human-machine interactions?
- The LLMs' failure to commit to explanations may lead to negative affect in human conversational partners, but could also foster curiosity.
- Understanding how the LLMs' performance on mentalistic inferences (or their absences) influences human social cognition in dynamic interactions is an important area for future research.
3. What are the key recommendations for future research on machine theory of mind?
- The importance of systematic testing using a battery of theory of mind measures, exposing models to multiple sessions and variations, and comparing to human performance.
- The value of open access to LLM parameters, data, and documentation to allow targeted probing and experimentation informed by comparisons with human data.