
Breaking news: Scaling will never get us to AGI
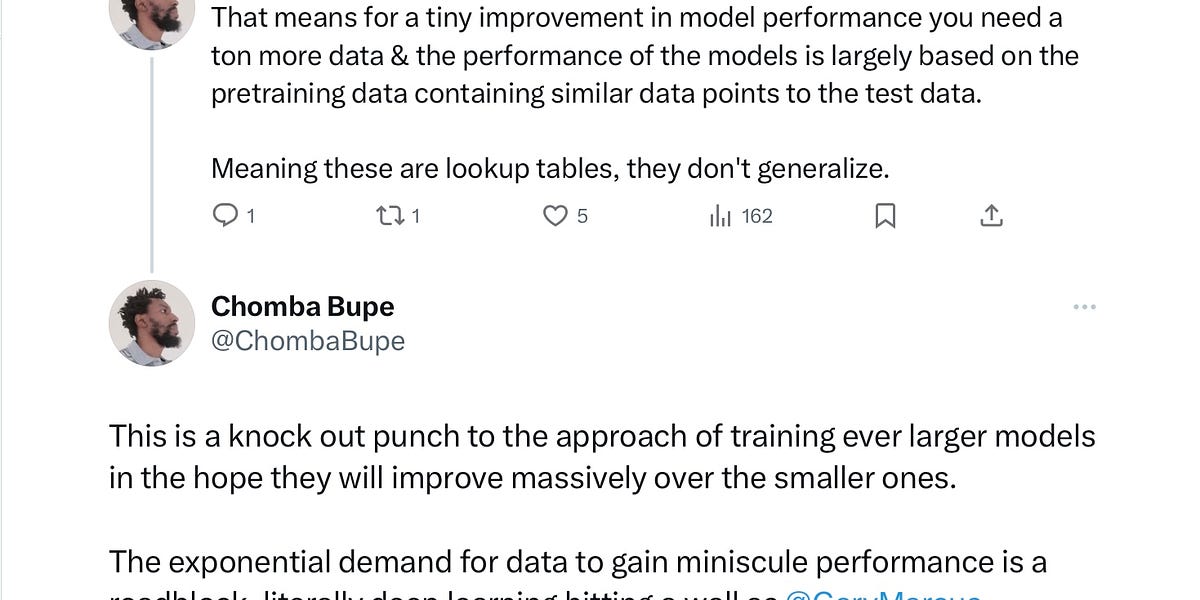
๐ Abstract
The article discusses the limitations of current neural network models in achieving general artificial intelligence (AGI), arguing that scaling up these models will not be sufficient to reach AGI. It highlights the inability of neural networks to generalize beyond their training data, which restricts their reasoning and planning capabilities. The article suggests that alternative approaches are needed to achieve AGI, as the exponential demand for data will eventually exceed what is available.
๐ Q&A
[01] Breaking news: Scaling will never get us to AGI
1. What are the key limitations of current neural network models in achieving AGI?
- Neural networks (at least in the configurations that have been dominant over the last three decades) have trouble generalizing beyond the multidimensional space that surrounds their training examples
- This limits their ability to reason and plan reliably
- It also drives their greediness with data and the ethical choices their developers have been making
- There will never be enough data, and there will always be outliers
2. Why are driverless cars still just demos, and why will LLMs never be reliable?
- The inability of neural networks to generalize beyond their training data is the reason why driverless cars are still just demos and why LLMs will never be reliable
3. What is the author's proposed solution to achieve AGI?
- To get to AGI, we need alternative approaches that can generalize better beyond the data on which they have been trained
[02] An important new preprint that just came out on data and scaling
1. What does Chomba Bupe, the tech entrepreneur and computer vision researcher, explain about the new preprint?
- In short, diminishing returns are what we can expect as we scale up neural network models
- Current models aren't literally look-up tables, they can generalize to some degree, but not enough
- They can generalize within a space of training examples but face considerable trouble beyond that space
2. What is the author's view on the implications of this new preprint?
- Sooner or later, the exponential greed for data will exceed what is available
- To get to AGI, we need alternative approaches that can generalize better beyond the data on which they have been trained
[03] Response to the author's argument
1. What is the commenter's view on the author's argument about the reliability of LLMs?
- The commenter agrees with the technical sentiment that LLMs, when overfitted, untuned, and unaugmented with other vectors, are not reliable to use in general and not intelligent
- The commenter suggests that using a bell curve when a flow chart would make more sense logically for some of these LLM wrapper type apps
2. What is the commenter's view on the author's argument about the need for alternative approaches to achieve AGI?
- The commenter agrees that we need more than just LLMs to achieve AGI
- The commenter argues that AI has been a bunch of non-LLM, non-transformer type AI technology that doesn't rely on pretrained models, and that we already have AGI, even if it's not superintelligent
- The commenter summarizes the author's argument as "I wish AGI wasn't so general that it's unreliable. Now let's do some real AI stuff besides just pretrained transformers ad nauseum."
3. What evidence does the commenter provide to support the reliability of driverless cars?
- The commenter provides a link to YouTube videos showing practically flawless driving by FSD 12.3, suggesting that driverless cars are already reliable.