
Why reliable AI requires a paradigm shift
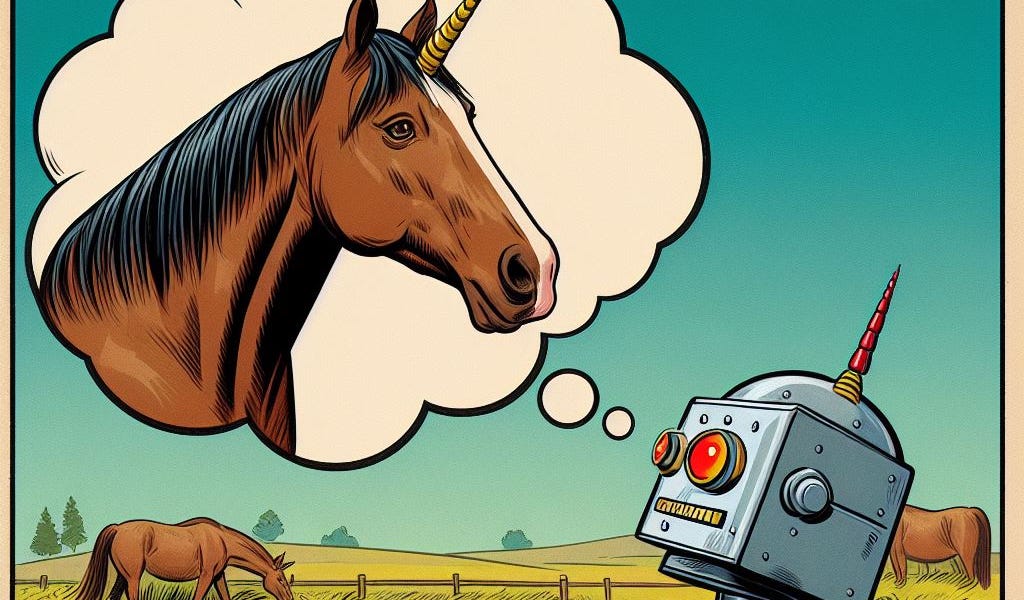
๐ Abstract
The article discusses the fundamental issue of hallucinations in AI systems, particularly large language models (LLMs), and the need for a paradigm shift to address this challenge.
๐ Q&A
[01] Why reliable AI requires a paradigm shift
1. What are hallucinations in the context of AI systems?
- Hallucinations refer to the phenomenon where an AI model generates outputs that appear plausible and coherent but do not accurately reflect real-world facts or the system's intended purpose.
- These hallucinations can manifest in various forms, such as fabricated information, nonsensical responses, or outputs that are inconsistent with the input data.
- Hallucinations can occur in various AI applications, from natural language processing to computer vision and beyond.
2. How are hallucinations different from other typical failures of generative models?
- Out-of-distribution errors occur when an AI model is presented with input data significantly different from its training data, causing it to produce unpredictable or nonsensical outputs.
- Biased outputs arise when an AI model's training data or algorithms contain inherent biases, leading to the generation of outputs that reflect those biases.
- Hallucinations involve the AI model generating information that is not necessarily biased but completely fabricated or detached from reality, making them more insidious and difficult to detect.
3. What are the real-world implications of AI hallucinations?
- Hallucinations in AI systems, particularly in high-stakes applications like healthcare, finance, or public safety, can have significant consequences, leading to erroneous decision-making, false conclusions, and potentially harmful outcomes.
- Even in low-stakes applications, the insidious nature of hallucinations makes them a fundamental barrier to the widespread adoption of AI, as it becomes virtually impossible for end-users to detect and correct the errors.
- Developing and deploying LLMs with hallucination capabilities raises critical ethical considerations, requiring responsible AI development practices that prioritize transparency, accountability, and the mitigation of potential harms.
4. Why do hallucinations happen in current generative AI models?
- The underlying cause of hallucinations in large language models is that the current language modeling paradigm used in these systems is, by design, a hallucination machine.
- Generative AI models rely on capturing statistical patterns in their training data to generate outputs, rather than having a clear, well-defined understanding of what is true or false.
- The statistical nature of language models makes them susceptible to hallucinations, as they implicitly assume that small changes in the input (the sequence of words) lead to small changes in the output (the probability of generating a sentence), even if the sentence is factually incorrect.
- The smoothness hypothesis, which is necessary for computational convenience, also contributes to the inherent tendency of these models to hallucinate, as they cannot define a crisp frontier between true and false sentences.
5. Can hallucinations be completely eliminated in the current paradigm of generative AI?
- No, hallucinations cannot be completely eliminated in the current paradigm of generative AI, as they are an inherent feature of how these models work.
- Even if the probability of hallucinations can be pushed to a sufficiently low value, recent research suggests that there will always be a prompt that can generate a hallucinated output with almost 100% certainty, making it impossible to guarantee the absence of hallucinations.
[02] Mitigating Hallucinations in AI
1. What are some strategies for mitigating hallucinations in AI systems?
- Incorporating external knowledge bases and fact-checking systems into the AI models to ground them in authoritative, verified information sources.
- Developing more robust model architectures and training paradigms less susceptible to hallucinations, such as increasing model complexity, incorporating explicit reasoning capabilities, or using specialized training data and loss functions.
- Enhancing the transparency and interpretability of AI models to make their decision-making processes more transparent and easier to identify and rectify the underlying causes of hallucinations.
- Establishing standardized benchmarks and test sets for hallucination assessment to quantify the prevalence and severity of hallucinations and compare the performance of different models.
- Fostering interdisciplinary collaboration between AI researchers, domain experts, and authorities in related fields to advance the understanding and mitigation of hallucinations.
2. Why is a paradigm shift necessary to address the issue of hallucinations in AI?
- The article suggests that the current language modeling paradigm used in generative AI systems is, by design, a hallucination machine, as it lacks a clear, well-defined understanding of what is true or false and relies on statistical patterns in the training data.
- Incremental improvements to the current technology may not be sufficient to solve the issue of hallucinations entirely, and a new machine learning paradigm altogether may be necessary to address the fundamental limitations of the existing approaches.
Shared by Daniel Chen ยท
ยฉ 2024 NewMotor Inc.