
How to Grade AI (And Why You Should)
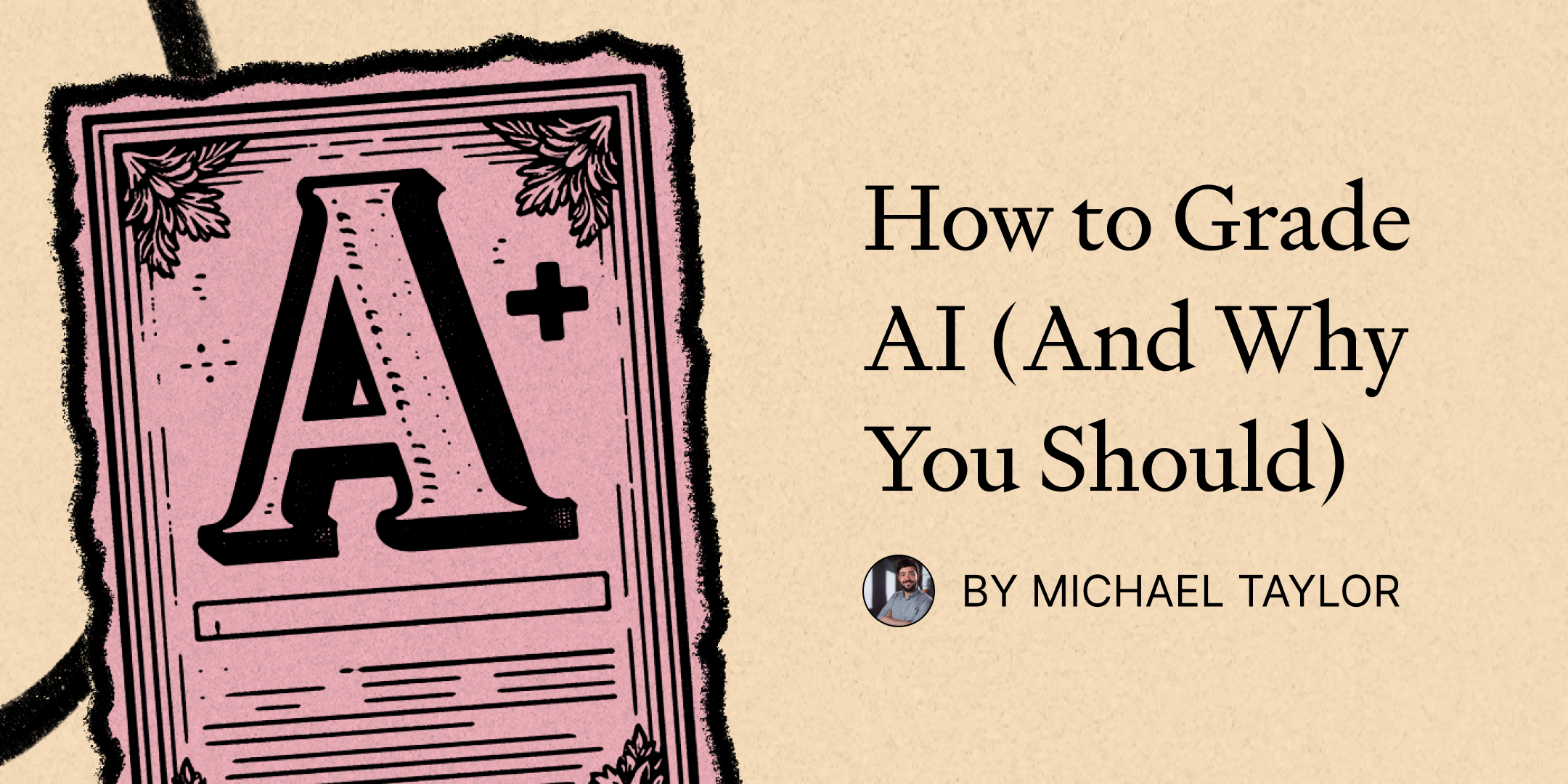
๐ Abstract
The article discusses the importance of evaluation metrics (evals) in the field of prompt engineering for AI systems. It covers the different types of evals (programmatic, synthetic, and human) and their strengths and weaknesses, as well as provides examples of how the author has used these evals in their work.
๐ Q&A
[01] Importance of Evals
1. Why are evals so important in prompt engineering for AI systems?
- Evals are how we measure the alignment between AI responses and business goals, as well as the accuracy, reliability, and quality of AI responses.
- Evals are matched against benchmarks to help discern a model's quality and progress from previous models.
- Without evals, it's difficult to do the work of optimizing and improving AI prompts.
2. What are the three main categories of evals?
- Programmatic (rules-based) evals
- Synthetic (AI grading other AI results) evals
- Human (manual ratings) evals
3. What are the strengths and weaknesses of each type of eval?
- Programmatic evals are fast and inexpensive, but struggle with complex or subjective tasks.
- Synthetic evals using AI evaluators are cheaper than human evaluators, but can be unreliable and have latency issues.
- Human evals provide valuable ground truth, but can be tedious and time-consuming.
4. How does the author use a combination of these evals in their work?
- The author typically uses all three types of evals in their AI projects to triangulate the right answer.
- They emphasize the importance of having at least one programmatic metric to quickly identify wrong answers.
- They also highlight the value of using human feedback as "ground truth" to validate and train other evals.
[02] Challenges in Implementing Evals
1. What are some of the challenges the author has faced in implementing evals?
- Clients often struggle to define "good" in a way that can be automated or delegated, leading to slow progress and internal disagreements.
- Developing robust programmatic evals requires technical expertise and tailoring to the specific task at hand.
- Synthetic evals using AI evaluators can be costly and have latency issues.
- Human evals can be tedious and time-consuming, and there is evidence of outsourced workers using ChatGPT to complete these tasks.
2. How does the author address these challenges?
- The author emphasizes the importance of building a "basket" of eval metrics to triangulate the right answer.
- They highlight the strategic advantage of owning unique data and using evals to fine-tune custom AI models.
- The author also notes that providing clients with concrete numbers on performance improvements can help win them over.
Shared by Daniel Chen ยท
ยฉ 2024 NewMotor Inc.