
How to talk to someone who doesn't trust AI
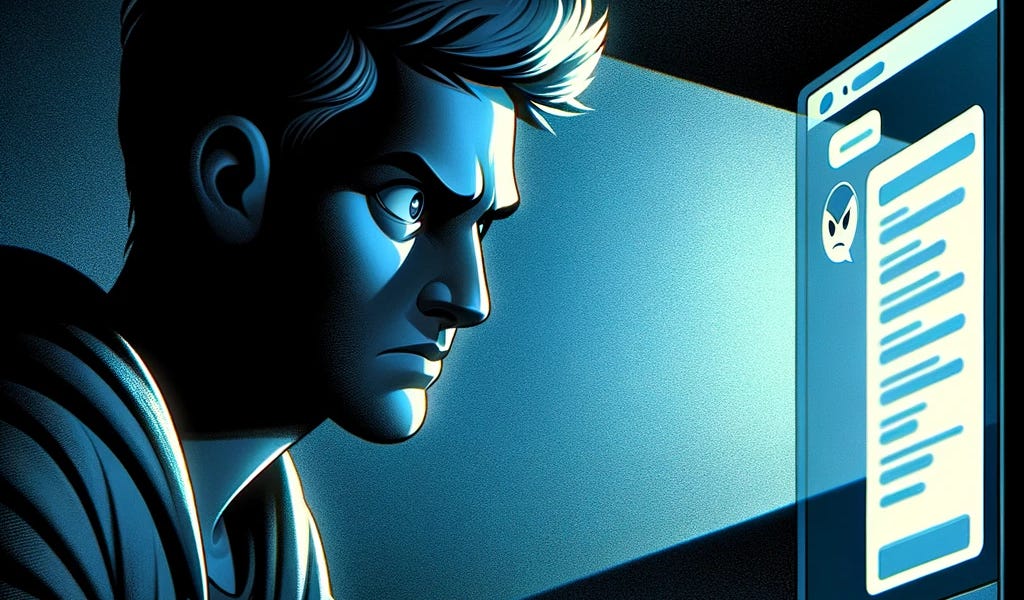
๐ Abstract
The article discusses how to talk to someone who doesn't trust AI, particularly those who are skeptical about the capabilities of large language models (LLMs). It covers common objections and provides counterarguments to address these concerns.
๐ Q&A
[01] "It's a great demo, but it doesn't actually work"
1. What is the common reason behind the skepticism that LLMs don't actually work? The skepticism is often driven by an understanding of pre-LLM machine learning techniques, where models were narrowly trained to accomplish a particular task rather than being general-purpose. This makes it seem unlikely that an LLM can coherently speak on a wide variety of topics, especially when given good data.
2. How can one counter this argument? The counterargument is to get the skeptics to try using an LLM, such as ChatGPT, to experience firsthand what it can do. This usually surprises them and gives them a sense of the capabilities of LLMs, from reciting general knowledge to identifying when they are wrong.
[02] "Look, it can't answer my question!"
1. What is the common objection behind this argument? The skeptic is trying to prove that if the technology was all that it was cracked up to be, it should be able to deal with every question it sees, no matter how obscure or vague the question might be. If the LLM cannot answer a specific question, the skeptic sees it as a "gotcha" moment to discredit the entire technology.
2. How can one counter this argument? The counterargument is that this is a misunderstanding of the core technology. LLMs have limitations, and identifying one of them is not surprising. The fact that an LLM gracefully bows out of answering a question is much better than if it were to hallucinate. LLMs are probabilistic tools that will occasionally make mistakes, just like humans, but that does not reduce the value of the other work they can do.
[03] "Every response from a chatbot looks the same"
1. What is the concern behind this argument? This critique usually comes later, and there is some validity to it. It's easy to tell if an LLM gets the first president of the US wrong, but once you get into more nuanced topics, it's genuinely difficult to know whether the nuances of the LLM's response are real facts or hallucinated nonsense. This is exacerbated by the fact that most LLMs are extremely verbose, making it hard to distinguish between genuine and generic-seeming responses.
2. How can one counter this argument? There is no general-purpose solution to this problem. Every AI-powered product needs to focus on customizing its answers based on its users' priorities and giving them control to customize what they see. The easiest thing to do is to reduce verboseness and increase answer quality, but this is easier said than done and requires time and thoughtful product UX.
[04] Overcoming skepticism
1. What is the key to converting skeptics? Converting skeptics means moving from vibes-based evaluations to empirically understanding the value LLMs are creating, which requires empirical evaluations. This means finding better ways to quantify model and application quality, as this will be critical to convincing the staunchest of the skeptics. If the impact of what is being built cannot be quantified, it will be difficult to close deals.