
Verbal lie detection using Large Language Models - Scientific Reports
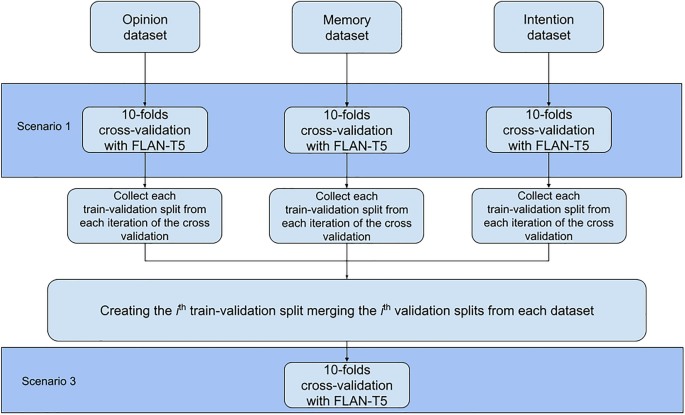
๐ Abstract
This study explores the performance of a Large Language Model, FLAN-T5 (small and base sizes), in a lie-detection classification task across three English-language datasets encompassing personal opinions, autobiographical memories, and future intentions. The study performs stylometric analysis to describe linguistic differences in the three datasets, and tests the FLAN-T5 model in three scenarios using 10-fold cross-validation. The results reveal that the model's performance depends on its size, with larger models exhibiting higher performance. The study also finds that linguistic features associated with the Cognitive Load framework may influence the model's predictions.
๐ Q&A
[01] Descriptive Linguistic Analysis
1. What were the key findings from the descriptive linguistic analysis comparing the three datasets? The descriptive linguistic analysis found that the linguistic features distinguishing truthful from deceptive statements varied across the three datasets (opinions, memories, intentions) in terms of:
- The number and type of significant linguistic features
- The magnitude of the effect sizes
- The direction of the effects
For example, truthful opinions were characterized by greater complexity, verbosity, and authenticity, while deceptive opinions showed higher concreteness and more other-references. Truthful memories had higher scores in memory-related words and contextual details, while deceptive memories had more cognitive words. Truthful intentions contained more concrete and distinct information about the intended action, while deceptive intentions had more cognitive words and were temporally oriented toward the present and past.
2. How did the results support Hypothesis 5a? The results from the descriptive linguistic analysis supported Hypothesis 5a, which stated that the linguistic style distinguishing truthful from deceptive statements varies across different contexts. The analysis found significant differences in the linguistic features that differentiated truthful and deceptive statements across the three datasets, confirming that the linguistic style of deception is context-dependent.
[02] Lie Detection Task
1. What were the key findings from testing the FLAN-T5 model in the three scenarios?
- Scenario 1 (fine-tuning and testing on a single dataset): The FLAN-T5 model achieved state-of-the-art results, outperforming previous benchmarks.
- Scenario 2 (fine-tuning on two datasets, testing on the third): The model performed at chance level, suggesting it requires exposure to prior examples to accurately classify deceptive texts within different domains.
- Scenario 3 (fine-tuning and testing on the aggregated datasets): The FLAN-T5 base model achieved the highest accuracy, outperforming the smaller FLAN-T5 model. This suggests model performance depends on size, with larger models showing higher accuracy.
2. How did the results compare to classical machine learning and deep learning approaches? The FLAN-T5 model outperformed the classical machine learning baseline (bag-of-words + logistic regression) and previous deep learning approaches on the same datasets. The authors attribute this to the FLAN-T5 model's ability to leverage its robust language representation and adapt it to the lie detection task through fine-tuning, which is more effective than training simpler models from scratch.
3. What was the purpose of the explainability analysis, and what were the key findings? The explainability analysis aimed to investigate whether the linguistic style of the input statements influenced the FLAN-T5 base model's predictions. The analysis compared correctly classified and misclassified statements, finding that correctly classified statements shared linguistic features related to the Cognitive Load theory, such as length, complexity, and analytical style. In contrast, truthful and deceptive misclassified statements did not exhibit significant differences in linguistic style. This suggests the model's performance was influenced by the linguistic cues associated with cognitive load, which may be more generalizable across the different deception contexts.
</output_format>