
Lessons from customer evaluations of an AI product
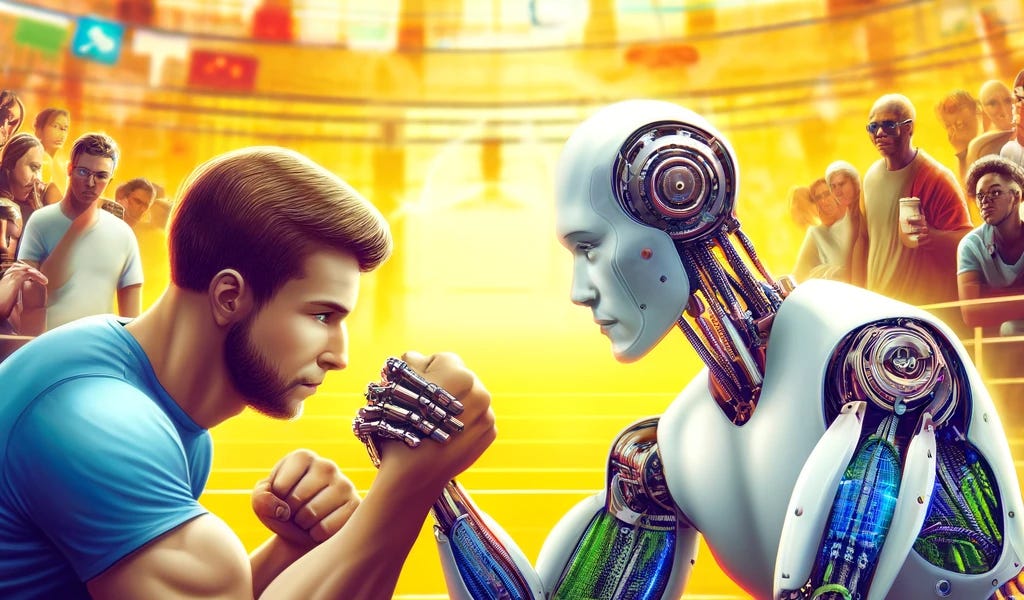
๐ Abstract
Lessons learned from customer evaluations of an AI product, RunLLM, including trends in how customers evaluate early-stage AI products, the importance of data quality, managing customer expectations, and the role of "vibes-based" evaluations.
๐ Q&A
[01] Lessons from Customer Evaluations
1. What are some key trends the authors have observed in how customers evaluate early-stage AI products?
- Customers who have prior experience building custom AI assistants tend to be better at evaluating the product, as they understand failure modes and can recognize higher quality responses more quickly.
- These experienced customers often come with pre-defined evaluation sets to baseline their expectations.
- Customers without prior AI assistant experience tend to rely more on "vibes-only" evaluations.
2. Why is evaluating LLMs and LLM-based products particularly challenging?
- Unlike evaluations of traditional software like CRMs or databases, there are no set criteria or feature matrices for evaluating LLM-based products.
- The authors note that "we're all making it up as we go" when it comes to evaluating these types of AI products.
3. What is the importance of data quality in the performance of the AI product?
- The quality and specificity of the data used at every stage of the pipeline (data ingestion, fine-tuning, processing user inputs, generating responses) is the primary determinant of the quality of the AI's answers.
- When customers provide feedback on poor answers, the authors find that the root cause is usually a lack of necessary data, rather than a limitation of the technology.
[02] Managing Customer Expectations
1. What are the two main camps of people unhappy with AI products, and how do the authors approach each?
- The first camp are those who see AI as a "party trick" that only generates useful answers sometimes.
- The second camp are the "maximalists" who expect the AI to be able to impute answers from any information, even if it's not written down.
- The authors find it easier to convince the skeptical first camp, as the maximalists tend to blame the product builders for any errors, rather than understanding the limitations of the underlying technology and data.
2. How do the authors recommend addressing the challenge of managing customer expectations?
- The authors emphasize the need to clearly explain to customers that AI is a powerful tool, but its performance is still limited by the quality and completeness of the underlying data.
- They suggest developing product- and task-specific evaluation measures, rather than relying on generic benchmarks, to better surface the capabilities and limitations of the AI product to customers.
[03] The Role of "Vibes-Based" Evaluations
1. What is meant by "vibes-based" evaluations, and why do the authors consider them important?
- "Vibes-based" evaluations refer to the practice of simply trying out the AI product and assessing the quality of the responses based on intuition and subjective impressions.
- While not an empirical solution, the authors acknowledge that this is often the dominant evaluation method for LLM-based products.
- They note that customers' intuition about the types of questions their product should be able to answer is often quite good, and that much of the confidence (or lack thereof) in the product comes from these one-off "vibes-based" interactions.
2. What are the potential downsides of relying too heavily on "vibes-based" evaluations?
- Vibes-based evaluations can either sell the product short (by not realizing the need for more data) or oversell the product (by happening to ask questions the AI excels at, while missing the ones it struggles with).
- The authors suggest that as a still-forming market, AI product builders need to think about how to better evaluate their own tools and surface those insights to customers, rather than relying solely on "vibes-based" feedback.