
The AI Plateau Is Real — How We Jump To The Next Breakthrough
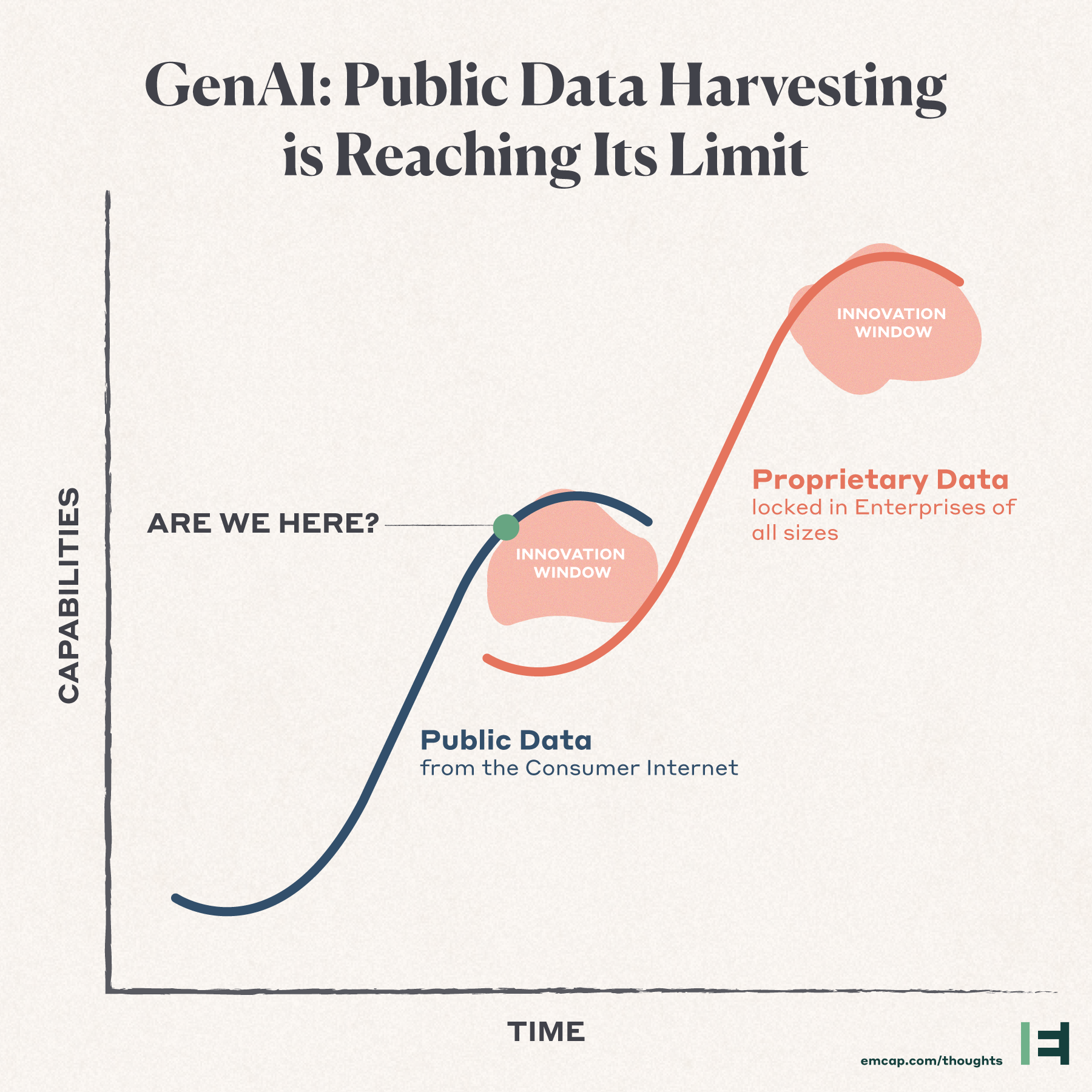
🌈 Abstract
The article discusses the current limitations and plateaus in the progress of generative AI models, and proposes that the next breakthrough in AI performance will come from leveraging high-quality data produced in the workplace context, rather than relying solely on public internet data. It outlines four key areas of opportunity for new startups to solve the AI plateau in a way that aligns with the needs and imperatives of businesses.
🙋 Q&A
[01] The S-Curve of Technological Advancement
1. What is the common pattern observed in technological advancements across centuries?
- The article states that technological advancement follows an S-Curve pattern, where an almost undetectable buildup of knowledge and craft is met with a spark, resulting in an explosion of innovation, and eventually reaching a plateau.
2. How does this pattern apply to the AI revolution?
- The article cites the example of the AI revolution, where the progress from Alan Turing's early explorations to the creation of large language models like ChatGPT by OpenAI follows this S-Curve pattern.
3. What is the current state of progress in large language models?
- The article notes that the pace of progress in large language model performance has slowed, with incremental improvements becoming more limited. It presents a chart showing the performance increases of OpenAI's flagship model, which indicates a flattening of the S-Curve.
[02] The Next Frontier of Data
1. What are the limitations of the current data sources used to train large language models?
- The article states that the public textual data available on the internet has been extensively harvested, forcing AI companies to explore other sources like transcribing YouTube videos or using low-cost human "labelers". However, these tactics are unlikely to provide the breakthrough needed to jump to the next S-Curve.
2. Why is data produced in a work context more valuable for training AI models?
- The article argues that data produced in a work context, such as product specifications, sales decks, or medical studies, is of far higher quality and value for training purposes compared to unverified public data like Wikipedia pages or Reddit posts. It also notes that this work data often comes from experts in their respective fields.
3. What is the potential value proposition of AI for businesses?
- The article compares the average revenue per user (ARPU) of top consumer apps to the per-seat price of select B2B apps, suggesting that the value proposition of AI for businesses is vast and largely untapped.
[03] The Need for Businesses to Own Their Models
1. What are the concerns regarding AI companies' access to businesses' proprietary data?
- The article expresses concerns that as AI companies start to tackle business use cases, enterprises may be at risk of having their proprietary and sensitive data, such as knowledge bases, internal processes, contracts, and PII, harvested by these AI companies, similar to how consumer data was harvested by companies like Facebook.
2. Why is it important for businesses to own their own proprietary models?
- The article argues that businesses should resist the big AI companies' appetite to harvest their proprietary data, as this data composes the sustainable competitive advantage for businesses. Owning their own models allows businesses to continuously improve while sustaining their competitive advantage.
[04] Opportunities for New Startups
1. What are the four key areas of opportunity identified for new startups to solve the AI plateau?
- The article identifies four key areas of opportunity:
- Novel ways to source high-quality AI training data from experts in each field
- Helping enterprises prepare their business app data for model training or inference
- Capturing net new data generated in enterprises' workflows without interruption
- Enabling enterprises to create and deploy their own custom models to stay in control and protect proprietary IP
2. Why are these areas fertile ground for new disruptors?
- The article states that these areas are experiencing a large market pull, making them fertile ground for new startups to solve the AI plateau in a way that aligns with the needs and imperatives of businesses.