
AI scaling myths
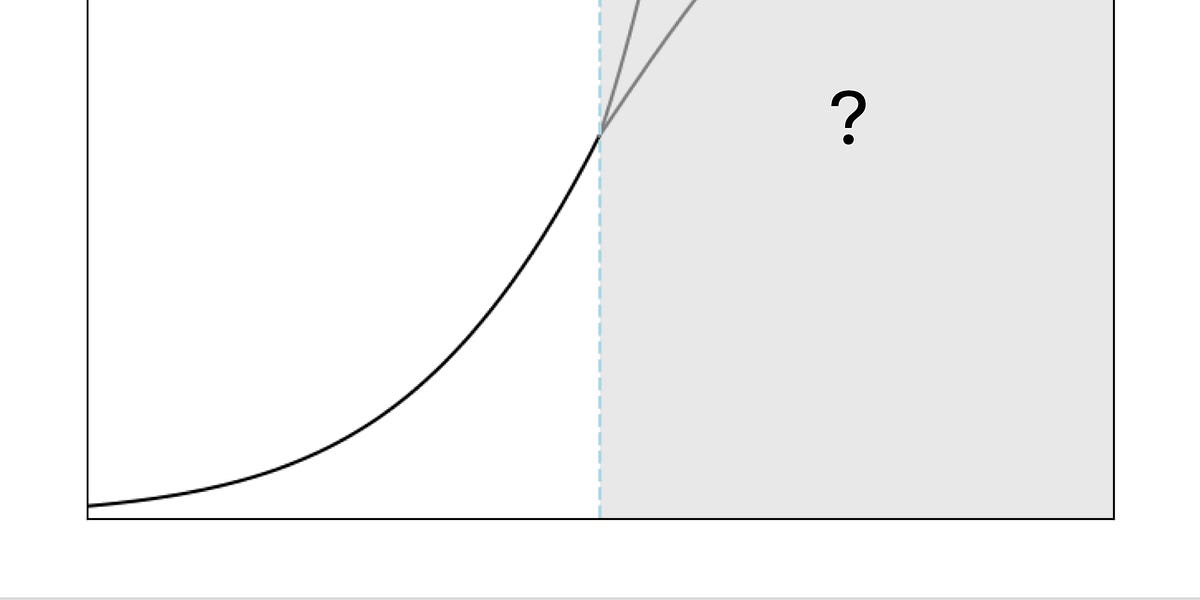
๐ Abstract
The article discusses the potential and limitations of scaling up language models (LLMs) to achieve artificial general intelligence (AGI). It examines the myths and misconceptions around the predictability of scaling, the challenges in obtaining high-quality training data, and the industry's shift towards smaller and more efficient models.
๐ Q&A
[01] Scaling Laws and Emergent Abilities
1. Questions related to the content of the section?
- What is the popular view on the potential of scaling up language models to achieve AGI?
- What are the misconceptions behind this view, according to the article?
- How do scaling laws quantify the improvement in language models, and why is this not the same as "emergent abilities"?
- Why might the trend of acquiring new capabilities through scaling not continue indefinitely?
Answers:
- The popular view is that the trends of increasing model size, training compute, and dataset size will continue and potentially lead to AGI.
- This view is based on a misunderstanding of what research on scaling laws has shown. Scaling laws only quantify the decrease in perplexity (improvement in predicting the next word), which is not the same as acquiring new "emergent abilities".
- There is no empirical regularity that gives confidence that the acquisition of new capabilities through scaling will continue indefinitely. The evidence suggests that LLMs may be limited in their ability to extrapolate beyond the tasks represented in their training data.
- At some point, having more data may not help further because all the tasks that are ever going to be represented in the training data are already represented. Traditional machine learning models eventually plateau, and LLMs may be no different.
[02] Barriers to Continued Scaling
1. What are the barriers to continued scaling of language models?
Answers:
- Obtaining high-quality training data is becoming increasingly challenging, as companies are already using all the readily available data sources. Expanding data sources like transcribing YouTube videos may not provide as much usable data as it might seem.
- There are also potential reputational and regulatory costs associated with data collection practices, as society might push back against certain data collection practices.
- The industry is already seeing a shift towards building smaller and more efficient models, as capability is no longer the primary barrier to adoption. Factors like cost, inference cost, and alignment are becoming more important.
- Training compute may continue to scale, as smaller models require more training to reach the same level of performance. However, this is a trade-off between training cost and inference cost.
[03] Synthetic Data and Self-Play
1. What are the limitations of using synthetic data to scale up language models? 2. How does the success of self-play in games like Go compare to its potential in more open-ended tasks?
Answers:
- The article suggests that the use of synthetic data is unlikely to have the same effect as having more high-quality human data. Synthetic data generation is more useful for fixing specific gaps and making domain-specific improvements, rather than replacing current sources of pre-training data.
- Self-play, as demonstrated in games like Go, is a successful example of "System 2 --> System 1 distillation", where a slow and expensive "System 2" process generates training data to train a fast and cheap "System 1" model. However, the article suggests that this strategy may be the exception rather than the rule for more open-ended tasks, such as language translation, where significant improvement through self-play is less likely.
[04] Implications for AGI
1. How do the authors view the history of AI progress and the concept of AGI? 2. What is the authors' perspective on recent claims about the plausibility of AGI by 2027?
Answers:
- The authors view the history of AI progress as a "punctuated equilibrium", which they call the "ladder of generality". They see instruction-tuned LLMs as the latest step in this ladder, with an unknown number of steps ahead before reaching a level of generality that could be considered AGI.
- The authors consider the recent essay claiming "AGI by 2027 is strikingly plausible" as an exercise in trendline extrapolation, which conflates benchmark performance with real-world usefulness. They note that many AI researchers have made the skeptical case about the timeline and capabilities of AGI.