
When Not to Use Generative AI
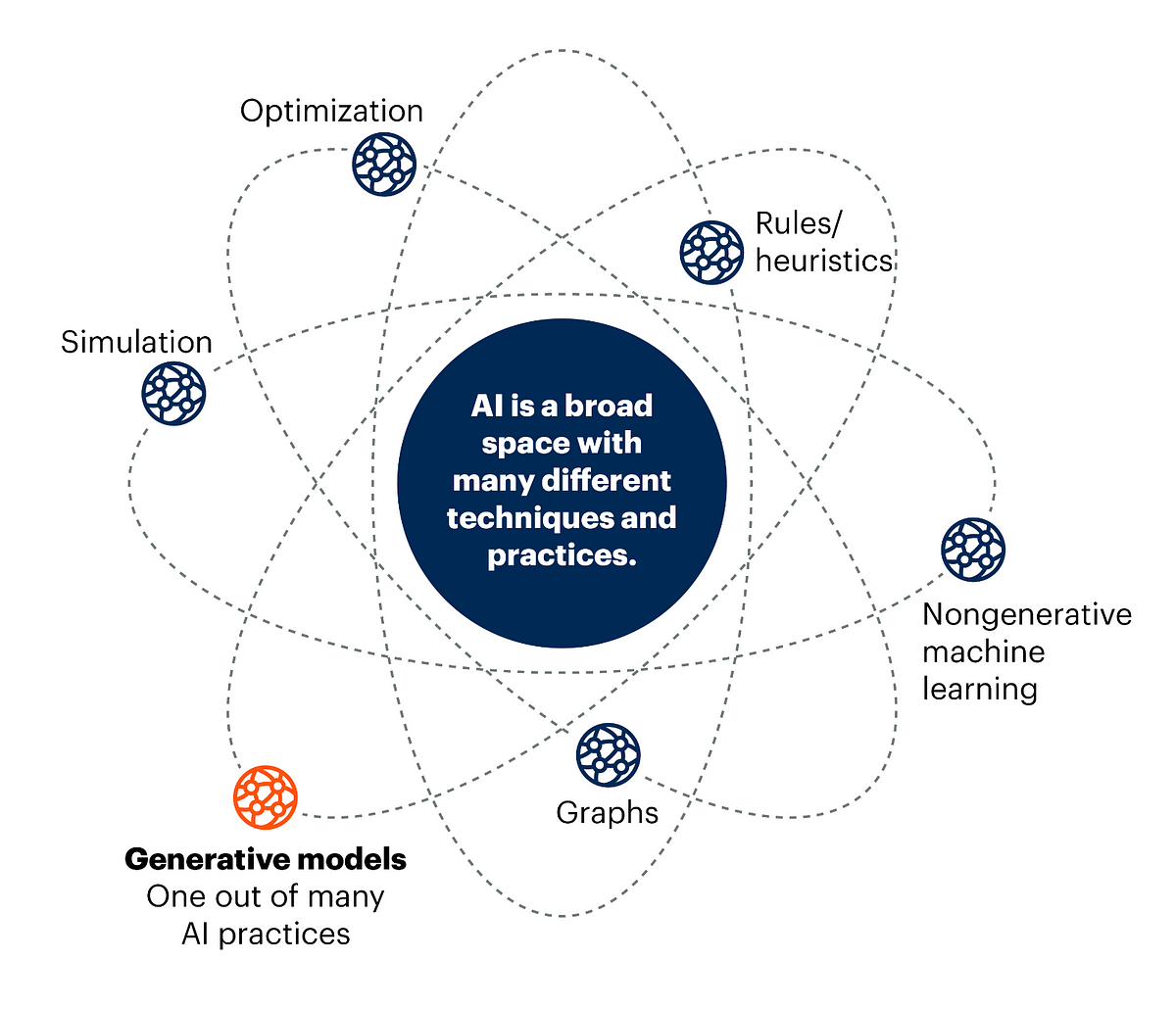
๐ Abstract
The article discusses the use of Generative AI (GenAI) in business and organizational contexts, highlighting the need to carefully assess its applicability and limitations. It emphasizes that GenAI is just one part of the broader AI landscape, and that a combination of different AI techniques may be required to effectively address business problems.
๐ Q&A
[01] Assessing the Applicability of GenAI
1. What are the key considerations when deciding whether to use GenAI or an alternative AI technique?
- Assess whether the use case is value-driving for the business and feasible to execute, regardless of the AI technique
- Recognize that many use cases may not actually need AI and do not merit further consideration
- Understand that GenAI is not a silver bullet and can be a poor fit in environments and cases where the common risks are unacceptable and cannot be effectively mitigated
2. What are some alternative AI techniques that can be considered instead of or in combination with GenAI?
- Standard established techniques include non-generative machine learning (ML), optimization, simulation, rules/heuristics, and knowledge graphs
- Emerging techniques such as causal AI, neuro-symbolic AI, and first-principles AI are also worth tracking
3. What are the benefits of trying a more straightforward alternative AI technique before diving into GenAI?
- They are often less risky, less expensive, and easier to understand
[02] Combining AI Techniques for Better Outcomes
1. What are the key insights from Gartner on aligning AI initiatives with strategic business objectives and financial performance metrics?
- Mature AI organizations can gain significantly from their AI initiatives if they align them closely with strategic, specific business objectives and financial performance metrics
- More than one AI approach and strategy will be required to do so effectively
2. What are some examples of strong combinations and use cases for different AI techniques?
- Combining techniques like nongenerative ML, optimization, and knowledge graphs can deliver better accuracy, transparency, and performance while reducing costs and the need for data