
Falling LLM Token Prices and What They Mean for AI Companies
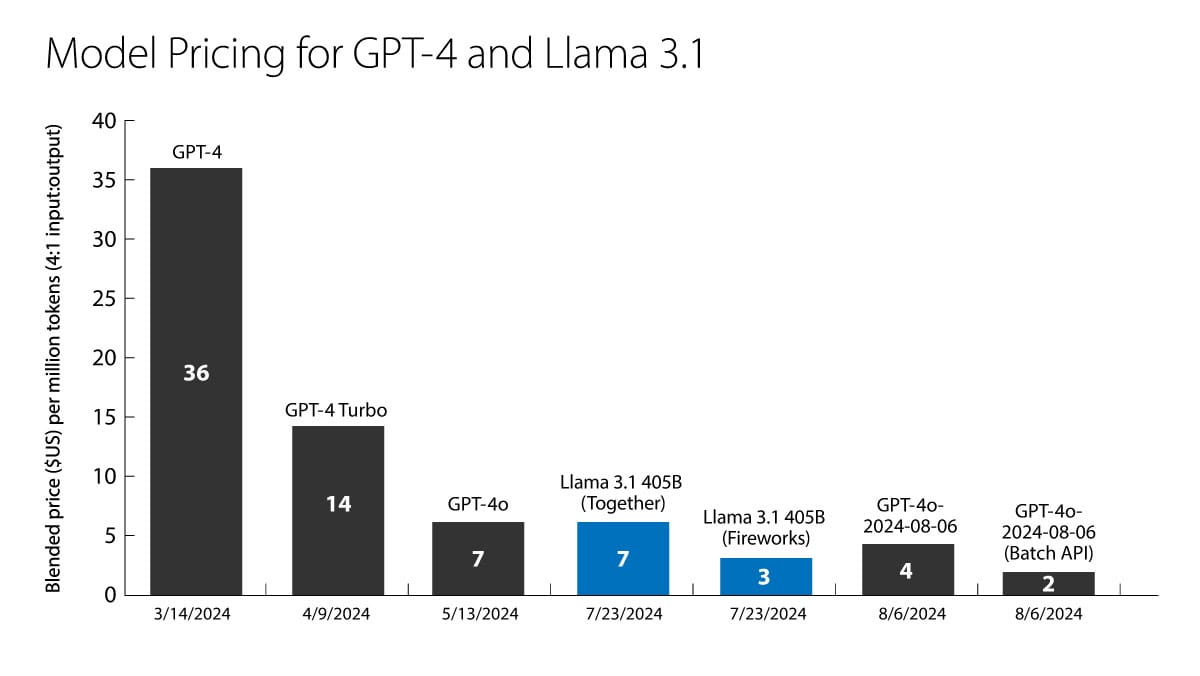
๐ Abstract
The article discusses the rapid decline in the cost of GPT-4 tokens, driven by factors such as the release of open-weight models like Llama 3.1, hardware innovations, and increased competition among API providers. It also explores the implications of falling token prices for building AI applications, particularly for agentic workloads, and provides recommendations for how AI companies can prepare for these changes.
๐ Q&A
[01] Recent Price Reduction and Implications
1. What is the current cost of GPT-4 tokens, and how does it compare to the initial release price?
- GPT-4 tokens now cost $4 per million tokens, down from $36 per million tokens at its initial release in March 2023, a 79% drop in price per year.
- OpenAI also offers a lower price of $2 per million tokens for using a new Batch API that takes up to 24 hours to respond to a batch of prompts, an 87% drop in price per year.
2. What are the key factors driving the rapid decline in token prices?
- The release of open-weight models like Llama 3.1, which allows API providers to compete directly on price and other factors without having to recoup the cost of developing a model.
- Hardware innovations from companies like Groq, Samba Nova, Cerebras, and the semiconductor giants, which are driving further price cuts.
3. How does the author recommend designing applications in light of the falling token prices?
- The author suggests designing applications based on where the technology is going, rather than only where it has been, as token prices are expected to continue falling rapidly.
- Even if an application's workload is not entirely economical now, falling token prices may make it economical at some point in the future.
[02] Preparing for Changing Token Prices
1. What are the challenges associated with switching between different model providers?
- While it may be possible to switch between providers of open-weight models like Llama 3.1 without too much testing, implementation details like quantization can lead to differences in model performance.
- A major barrier is the difficulty of implementing evals, which makes it challenging to carry out regression testing to ensure an application will still perform after swapping in a new model.
2. What is the author's outlook on the future of implementing evals and switching between models?
- The author is optimistic that as the science of carrying out evals improves, the process of switching between models will become easier.