
‘Time is running out’: can a future of undetectable deepfakes be avoided?
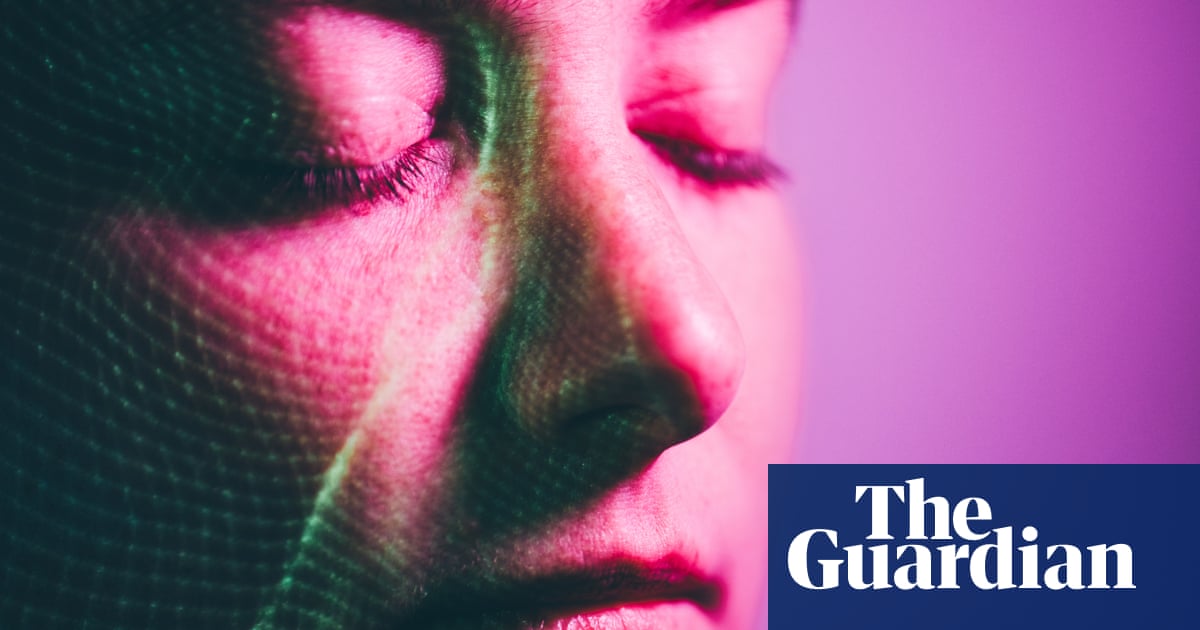
🌈 Abstract
The article discusses the challenges of detecting fake images generated by advanced AI models, as the technology continues to rapidly improve and become more accessible. It explores the race between detection and creation, and the limitations of current approaches like watermarking and labeling.
🙋 Q&A
[01] The Challenges of Detecting Fake Images
1. What are some of the manual techniques used to spot fake images, and why are they becoming less effective?
- Manual techniques like looking for misspelled words, incongruously smooth or wrinkly skin, and issues with hands and eyes can be used to spot fake images.
- However, these techniques are time-consuming and not scalable, as the AI models generating fake images are improving rapidly and making it increasingly difficult to detect them manually.
2. How are major tech companies and industry groups trying to address the issue of fake AI-generated content?
- The Coalition for Content Provenance and Authenticity, which includes companies like the BBC, Google, Microsoft, and Sony, has produced standards for watermarking and labeling AI-generated content.
- OpenAI has announced that it will adopt these standards for Dall-E 3, and Meta has started adding its own labels to AI-generated content.
- However, these labeling efforts have limitations, as not all AI content creators may adopt them, and labeling alone may not be enough to prevent the spread of misinformation.
[02] The Arms Race Between Detection and Creation
1. What are the challenges in automatically detecting AI-generated content?
- Automatically detecting AI-generated content is an ongoing challenge, with companies like Logically only achieving around 70% accuracy in their efforts.
- The problem is an "arms race" between detection and creation, as even AI generators without malicious intent will try to beat the detectors to create content that is as true to reality as possible.
2. What alternative approaches are being explored to address the issue of fake AI-generated content?
- Logically suggests looking at the behavior of disinformation actors, such as monitoring conversations on sites like 4chan and Reddit, and tracking the activity of suspicious accounts that may be co-opted by state actors.
- Other experts, like Ben Colman from Reality Defender, believe that while the fake side will continue to advance, there will always be the possibility of detection, even if it's just flagging something as possibly fake rather than reaching a definitive conclusion.
[03] The Broader Implications
1. How does the issue of fake AI-generated content go beyond just technical challenges?
- The article suggests that the problem of fake images goes beyond just the technical challenges of detection, as people's willingness to believe in such content is a significant factor.
- Even if state-of-the-art image generators are used to create fake content, the real issue is that people may still believe in it, regardless of the technical capabilities of detection.
2. What is the overall outlook on the future of detecting fake AI-generated content?
- The article concludes that while the fake side will continue to advance, the real side is not changing, and there will always be the possibility of detection, even if it's not a definitive conclusion.
- However, the article also suggests that this is just the start, and that addressing the broader societal issues around the willingness to believe in fake content will be crucial in the long run.