
Why Talk to Customers When You Can Simulate Them?
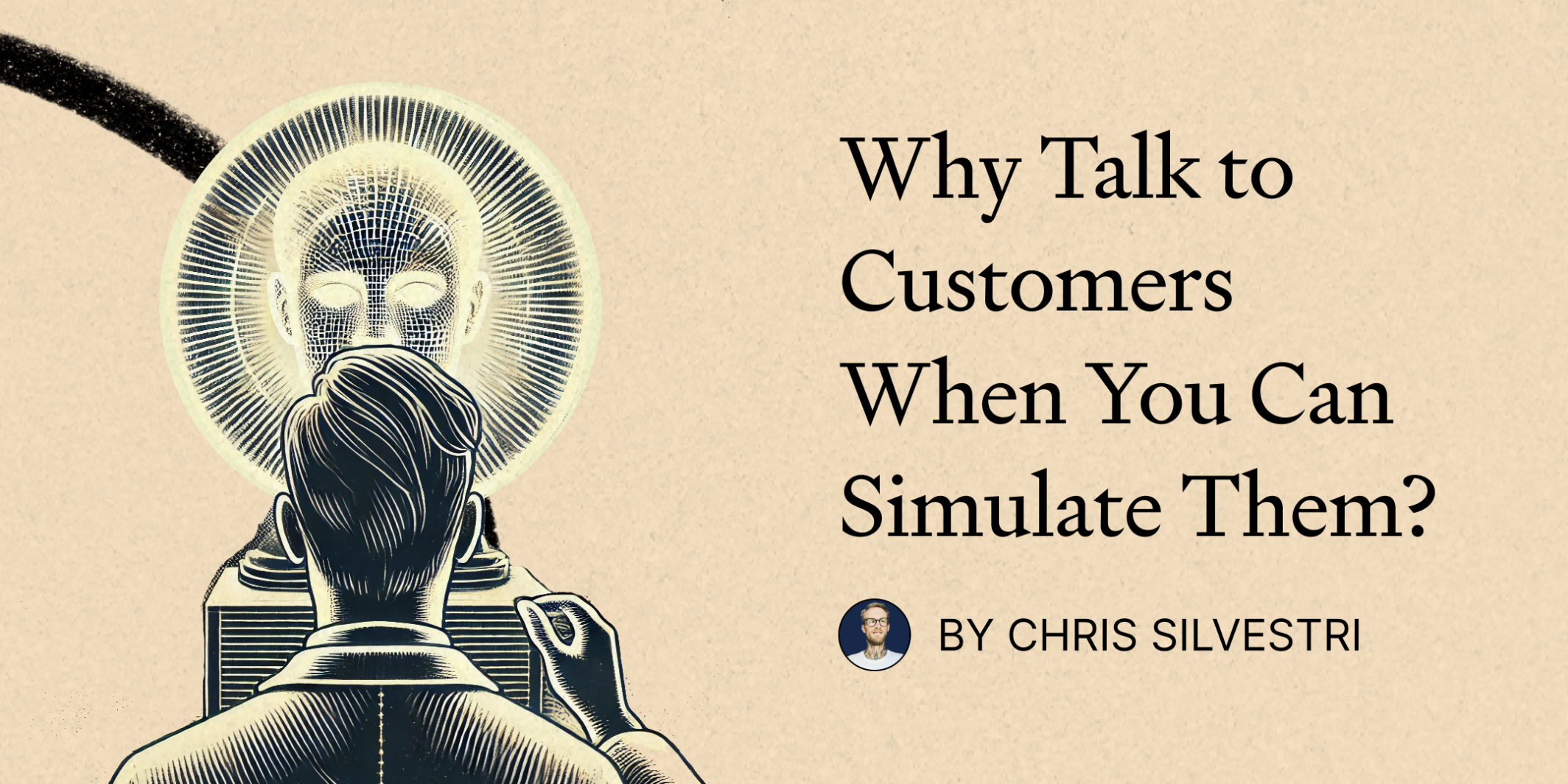
๐ Abstract
The article discusses the use of large language models (LLMs) for empathy engineering, a framework for using LLMs to better understand customers and create more effective marketing messaging. The key points covered include:
- The challenges of traditional market research and the potential of LLMs to provide faster and cheaper insights
- How to use LLMs to create detailed customer personas and simulate customer interactions to test marketing copy
- The importance of combining LLM-generated insights with human expertise and traditional research methods
- The benefits and limitations of using AI for customer research and marketing validation
๐ Q&A
[01] Empathy Engineering with LLMs
1. What is the problem that empathy engineering aims to solve?
- Empathy engineering aims to solve the problem of understanding what makes customers tick, which has plagued marketers and copywriters since the dawn of advertising.
- Traditional market research methods like customer interviews, review analysis, and A/B testing can be slow, expensive, and limited in their ability to uncover deep customer insights.
2. How does empathy engineering using LLMs work?
- Empathy engineering involves using LLMs to simulate conversations with detailed customer personas, allowing marketers to gain deeper insights into customer motivations, pain points, and decision-making processes.
- The process involves:
- Conducting thorough customer research to gather data
- Using the LLM to create detailed Ideal Customer Profiles (ICPs) based on the research
- Prompting the LLM to embody the customer persona and answer questions as if they were the actual customer
- Analyzing the LLM's responses to uncover insights for crafting more effective marketing messaging
3. What are the key benefits of using empathy engineering with LLMs?
- Speed and cost-effectiveness: Empathy engineering with LLMs can be done in a matter of hours for a few dollars, compared to the weeks and thousands of dollars required for traditional customer research methods.
- Deeper customer insights: LLMs can uncover nuances and connections in customer data that human researchers might miss, leading to more refined customer personas and messaging.
- Iterative testing: Marketers can quickly test multiple messaging variations with LLM-powered customer personas to identify the most effective copy.
[02] Limitations and Considerations of Empathy Engineering
1. What are the limitations and challenges of using LLMs for empathy engineering?
- LLMs can be unpredictable, with uneven capabilities and the potential to "hallucinate" or make up information.
- LLMs can be biased based on their training data, and their inner workings are not fully transparent.
- Empathy engineering requires a careful balance of using LLM-generated insights while still relying on human expertise and traditional research methods.
2. How can marketers mitigate the limitations of using LLMs for empathy engineering?
- Conduct thorough customer research to provide a strong foundation for the LLM-powered persona creation and testing.
- Continuously iterate and refine the LLM personas based on feedback from real-world customer interactions and testing.
- Maintain a human-in-the-loop approach, using the LLM as a powerful research assistant but not relying on it exclusively.
3. What is the role of human expertise in empathy engineering with LLMs?
- Human marketers and copywriters are still essential, as they can provide the necessary context, judgment, and creativity that LLMs lack.
- Combining LLM-generated insights with human expertise and traditional research methods is key to creating effective, authentic, and ethical marketing campaigns.
Shared by Daniel Chen ยท
ยฉ 2024 NewMotor Inc.