
AI companies are pivoting from creating gods to building products. Good.
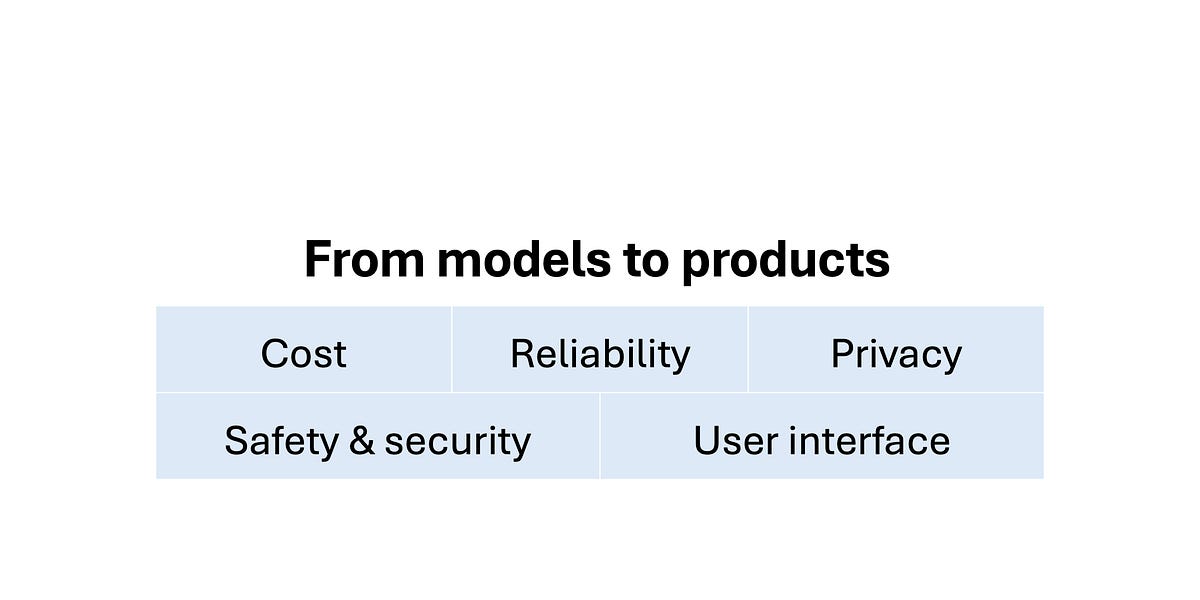
๐ Abstract
The article discusses the challenges and limitations that AI companies face in commercializing generative AI models, despite the significant investments being made in hardware and data centers. It examines the mistakes made by AI companies in their approach to commercialization and the barriers they still need to overcome to make generative AI commercially successful.
๐ Q&A
[01] Mistakes in Commercializing Generative AI
1. What were the two opposing but equally flawed approaches to commercializing LLMs?
- OpenAI and Anthropic focused on building models and not worrying about products, leading to a delay in releasing user-friendly apps.
- Google and Microsoft rushed to integrate AI into everything without thinking about which products would actually benefit from AI and how it should be integrated.
2. How did these approaches contribute to a poor public perception of the technology?
- The DIY approach of OpenAI and Anthropic meant that early adopters were more likely to be bad actors, as they were more invested in figuring out how to adapt the new technologies for their purposes.
- The AI-in-your-face approach by Microsoft and Google led to features that were occasionally useful but more often annoying, and caused unforced errors due to inadequate testing.
3. How are companies changing their ways?
- OpenAI is transitioning from a research lab focused on a speculative future to something resembling a regular product company.
- Anthropic has been picking up many of the researchers and developers at OpenAI who cared more about artificial general intelligence and felt out of place at OpenAI, but has also recognized the need to build products.
- Google and Microsoft are slower to learn, but Apple's slow and thoughtful approach to AI is likely to resonate more with users, and Google seems to have put more thought into integrating AI in its upcoming Pixel phones and Android.
- Meta's vision is to use AI to create content and engagement on its ad-driven social media platforms.
[02] Barriers to Commercializing Generative AI
1. What are the five limitations of LLMs that developers need to tackle to make compelling AI-based consumer products?
- Cost: Even in simple applications, cost concerns dictate how much history a bot can keep track of, and cost improvements can directly translate to accuracy improvements.
- Reliability: AI systems need to behave like deterministic software, but achieving perfect accuracy is intrinsically hard with statistical learning-based systems.
- Privacy: There are concerns around training models on sensitive user data and the potential for misuse of personal data by AI assistants.
- Safety and security: Unintentional failures, misuses of AI, and hacks that can leak user data or cause harm are significant concerns.
- User supervision: In many applications, there needs to be a way for the user to intervene if the bot goes off track, which is challenging with natural language interfaces.
2. Why are these challenges sociotechnical and not purely technical?
- Even if AI capability improves rapidly, developers have to solve the challenges discussed, which are not just technical but also involve integrating AI into existing products and workflows and training people to use it productively while avoiding its pitfalls.
- This process of integration and adoption is expected to happen on a timescale of a decade or more rather than a year or two.