
How to Choose Ideas for an LLM-powered Product to Thrive in a Fiercely Competitive Landscape
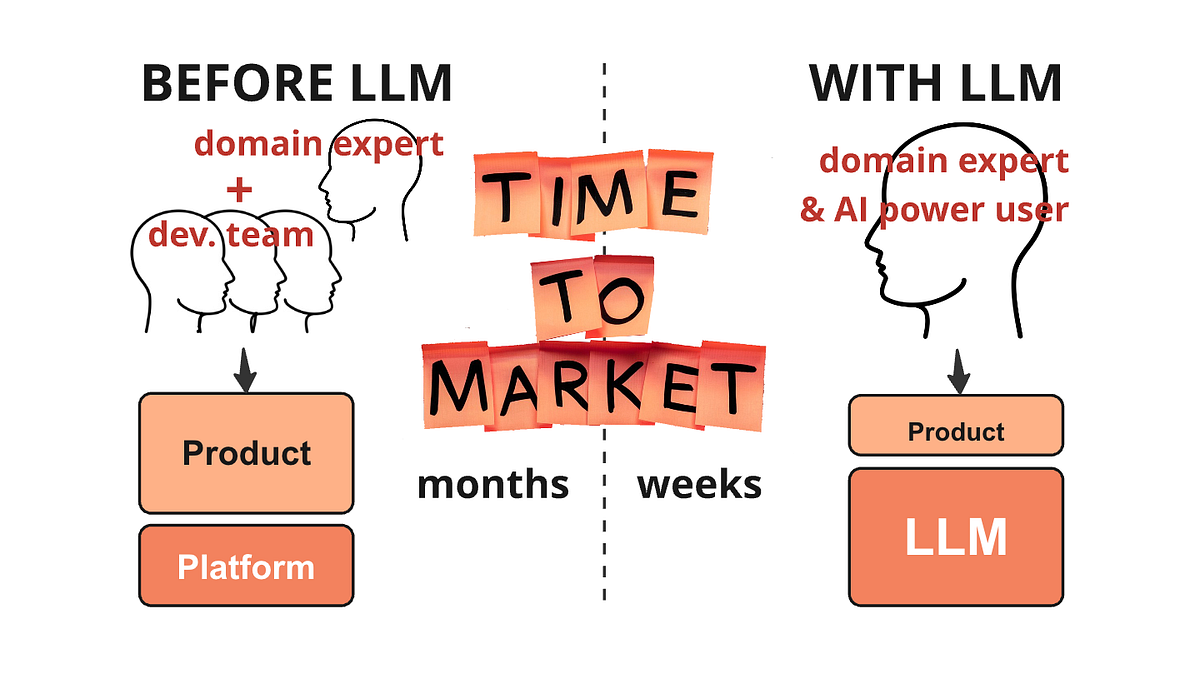
๐ Abstract
The article discusses strategies for developing successful GenAI (Generative AI) products, focusing on navigating the competitive landscape and optimizing development costs without losing competitive advantages. It covers key factors to consider when choosing MVP (Minimum Viable Product) scenarios, leveraging less obvious LLM (Large Language Model) capabilities, and the importance of domain expertise over technical expertise for GenAI product development.
๐ Q&A
[01] Navigating the Competitive Landscape and Optimizing Development Costs
1. What are the three main consequences of the rapid evolution of Generative AI?
- Rapid development cycles and short time-to-market periods are required for GenAI-based products.
- Frequent experimentation and rapid iteration are essential due to the unpredictability of LLM output and market response.
- Aligning with existing user goal-achievement patterns or industry leader trends is crucial for companies other than the largest industry leaders.
2. What types of LLM capabilities should be prioritized for MVP scenarios, and why?
- The author recommends focusing on LLM's less apparent capabilities, such as flipped interaction, contextual problem-solving, few-shot learning, and data analysis, rather than the more obvious capabilities like "creativity" and "answering any question."
- This approach saves resources on product delivery, as significant product value comes from the LLM itself rather than solely from the developers' efforts.
3. Why does the author advise against basing an MVP on LLM's ability to answer any question?
- The accuracy and quality of LLM's responses are inherently unpredictable, leading to potential issues.
- A product centered around question-answering cannot effectively compete with market leaders like ChatGPT.
[02] The Importance of Domain Expertise over Technical Expertise
1. Why does the author believe that domain expertise gains substantially more importance in the realm of Generative AI products?
- For LLM-based products, technical expertise plays a reduced role due to the easier software delivery, unlike traditional digital products where it's a crucial competitive advantage.
- A profound understanding of the domain becomes paramount, as this depth of knowledge is challenging for competitors to replicate.
2. How does the author suggest that the most efficient and lossless translation of domain expertise into technical implementation can occur?
- The author believes that when both business and technical visions reside in a single mind, the translation of domain expertise into technical implementation becomes more efficient.
- LLMs provide this opportunity by immensely reducing the technical expertise required for product implementation, enabling individuals with strong domain knowledge to take a direct role in product delivery.
3. What does the author suggest about the concept of an "LLM-driven one-person company"?
- The author is convinced that Generative AI will soon enable domain experts to single-handedly develop products within their domains, and this concept will be the focus of research in one of the author's upcoming articles.