
You can't build a moat with AI
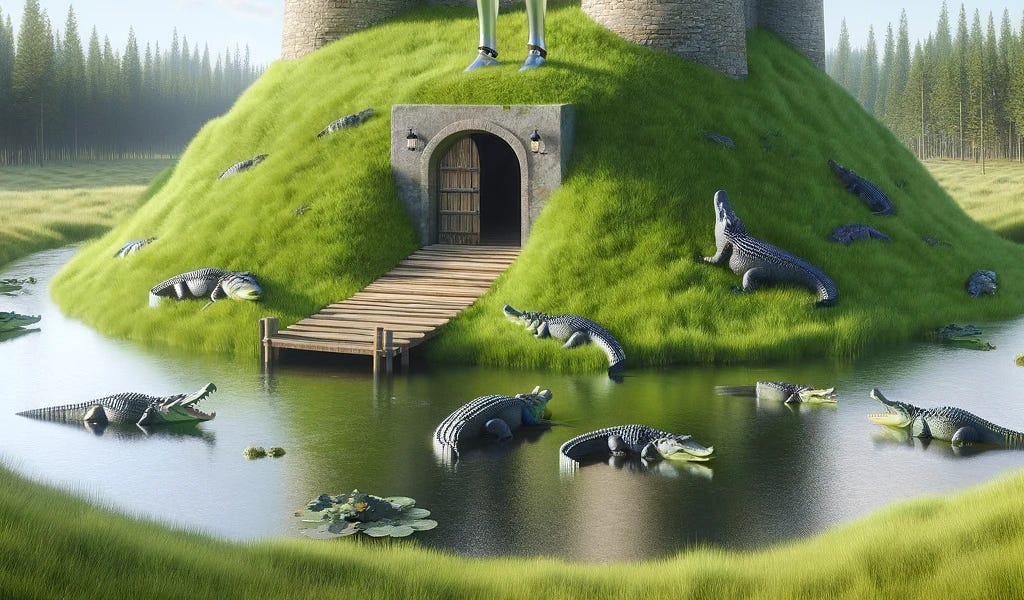
๐ Abstract
The article discusses the challenges of differentiating AI applications and the importance of data over language models (LLMs) in building successful AI applications.
๐ Q&A
[01] You Can't Build a Moat with AI
1. What are the key points made about differentiating AI applications?
- Differentiating AI applications is difficult, as everyone is using the same language models (e.g., GPT-4, Claude)
- The advantage of using the "right" language model is small, as they can be easily switched and experimented with
- Deviating from the norm with language models can be risky, as it may lead to unexpectedly good or bad results
- Prompts and prompt templates are not a good form of differentiation, as they can be quickly replicated by competitors
- The real differentiation lies in the data used to feed the language models
2. Why is the data more important than the language models?
- Language models are becoming commoditized, and the prompts are also easy to replicate
- The data used to feed the language models is what makes the real difference, as every customer/application will have slightly different data and preferences
- Proper data engineering and processing is crucial to ensure "garbage in, garbage out" is avoided
3. What is the author's view on the importance of AI expertise versus software engineering and data focus?
- Focusing too much on the AI aspect might be a detriment to differentiation at a certain point
- With thoughtful software engineering and a focus on customer data, a company can build a moat over time, even without being an "AI genius"
[02] Cynthia Systems Example
1. How does the Cynthia Systems example illustrate the importance of data over language models?
- Cynthia Systems has a powerful algorithmic moat that is unlikely to be replicated by competitors like Algolia and Bloomreach
- No language model like GPT-4 can achieve the same use cases as Cynthia Systems, as it can load and query an entire product catalog or music streaming app in real-time with guaranteed correctness
2. What is the author's view on the role of language models in this context?
- The author suggests that language models may become a "garbage collector of all the stupid things people want our LLM to do", implying that their usefulness is limited compared to the importance of data and data engineering.
Shared by Daniel Chen ยท
ยฉ 2024 NewMotor Inc.