
LINGO-2: Driving with Natural Language
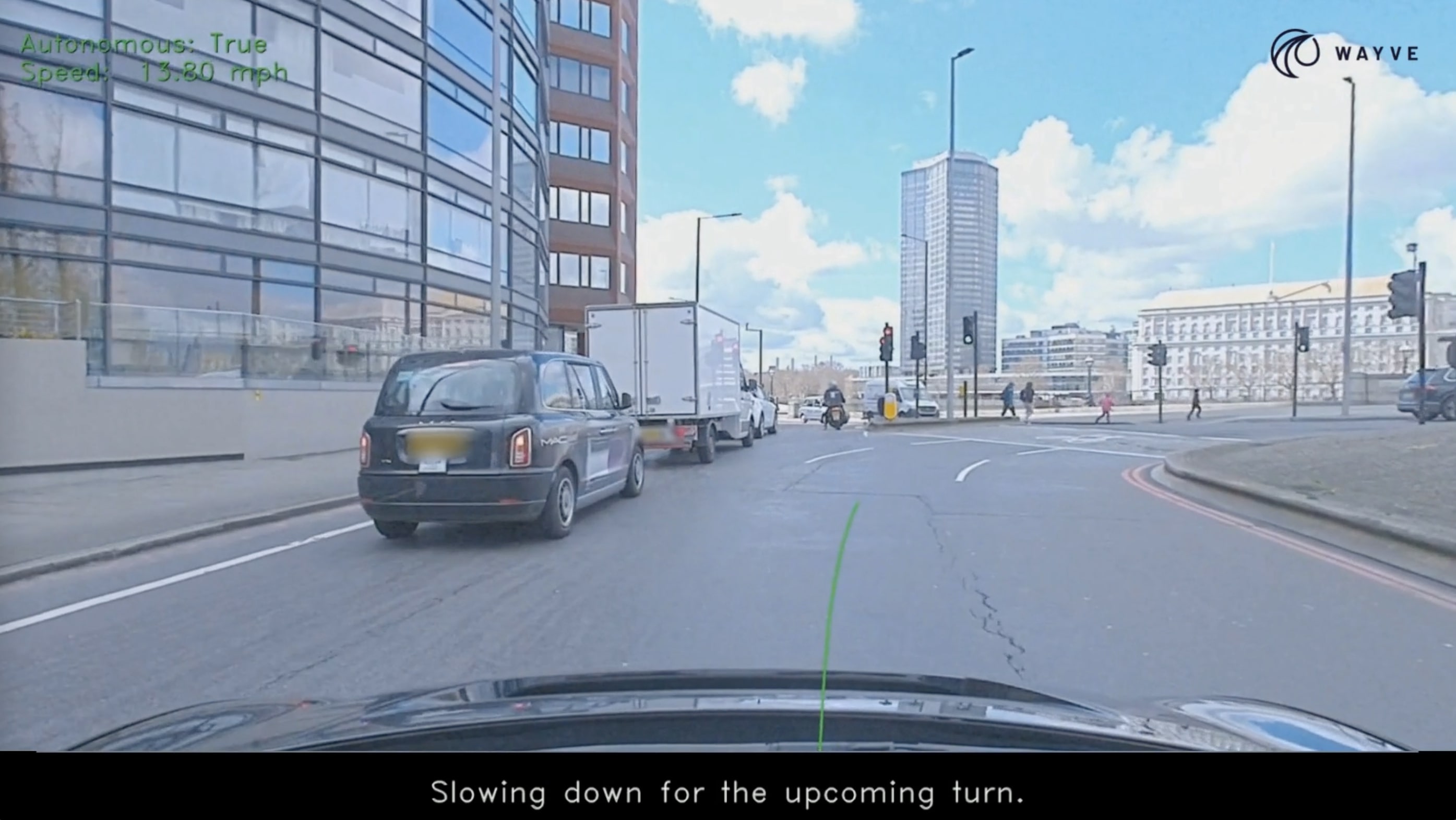
๐ Abstract
The article introduces LINGO-2, a closed-loop vision-language-action driving model (VLAM) that is the first driving model trained on language and tested on public roads. LINGO-2 combines vision and language as inputs and outputs, generating both driving actions and language to provide real-time driving commentary on its motion planning decisions.
๐ Q&A
[01] Introducing LINGO-2
1. What is LINGO-2 and how does it differ from the previous LINGO-1 model?
- LINGO-2 is a closed-loop vision-language-action driving model (VLAM) that combines vision and language as inputs and outputs, generating both driving actions and language to provide real-time driving commentary.
- In contrast, LINGO-1 was an open-loop driving commentator that leveraged vision-language inputs to perform visual question answering (VQA) and driving commentary, but its commentary was not integrated with the driving model.
2. What are the key capabilities of LINGO-2?
- LINGO-2 can adapt its driving behavior through language prompts, allowing the user to give commands or suggest alternative actions to the model.
- LINGO-2 can be interrogated in real-time, allowing the user to ask questions about the scene and the model's decisions while it is driving.
- LINGO-2 can provide real-time driving commentary, leveraging language to explain what it is doing and why, shedding light on the AI's decision-making process.
[02] LINGO-2 Architecture
1. What are the main components of the LINGO-2 architecture?
- LINGO-2 consists of two modules: the Wayve vision model and the auto-regressive language model.
- The vision model processes camera images of consecutive timestamps into a sequence of tokens, which are then fed into the language model along with additional conditioning variables.
- The language model is trained to predict a driving trajectory and commentary text, which is then executed by the car's controller.
[03] Adapting Driving Behavior through Linguistic Instructions
1. How can LINGO-2 adapt its driving behavior in response to language prompts?
- LINGO-2 can change its driving behavior in response to language prompts, such as "turning left, clear road," "turning right, clear road," or "stopping at the give way line."
- The model can also respond to prompts to either "stop behind the bus" or "accelerate and overtake the bus," as well as "continue straight to follow the route" or "slow down for an upcoming turn."
[04] Interrogating the AI Model in Real-time
1. How can LINGO-2 be interrogated in real-time?
- LINGO-2 allows the user to ask questions about the scene and the model's decisions while it is driving, such as "What is the color of the traffic lights?" or "Are there any hazards ahead of you?"
- The model can then provide real-time responses, explaining its observations and reasoning.
[05] Limitations
1. What are the current limitations of LINGO-2?
- More work is needed to quantify the alignment between the model's language explanations and its actual decision-making.
- Controlling the car's behavior with language in real-world settings needs to be investigated further to ensure reliability and safety, as the model should understand the context of human instructions while never violating appropriate limits of safe and responsible driving behavior.
Shared by Daniel Chen ยท
ยฉ 2024 NewMotor Inc.