
GPT-5: Everything You Need to Know
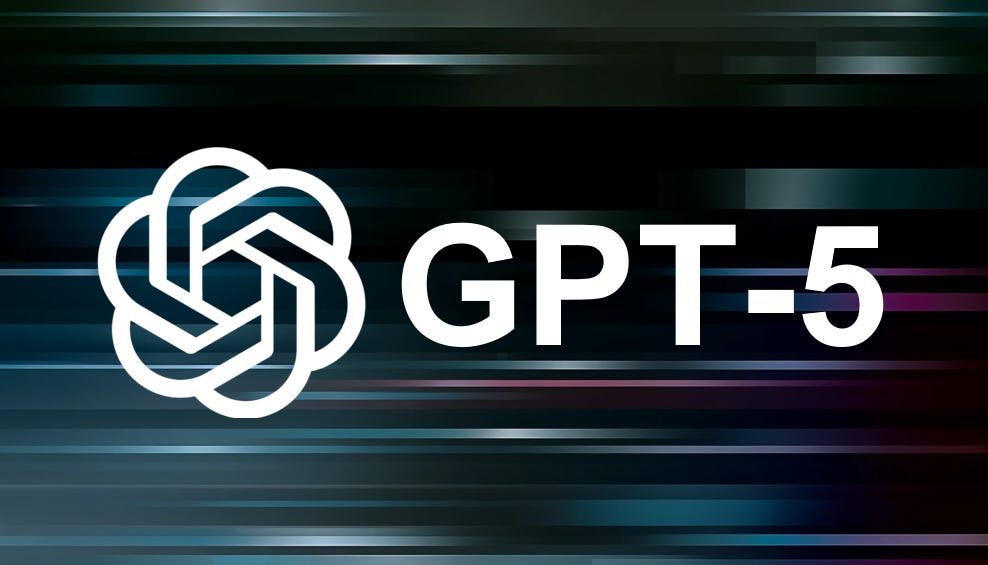
๐ Abstract
The article is a comprehensive analysis of the upcoming GPT-5 model from OpenAI, covering various aspects such as the competitive landscape, release timeline, technical capabilities, and potential breakthroughs. It also explores the broader implications of GPT-5 for the field of artificial intelligence.
๐ Q&A
[01] Some Meta About GPT-5
1. What are the key points discussed about the naming and release of GPT-5?
- The article suggests that the names "GPT-4.5" and "GPT-5" are arbitrary placeholders, as OpenAI is constantly improving its models and evaluating checkpoints to determine the appropriate release number.
- There are doubts about whether OpenAI will release a "GPT-4.5" model or go directly to "GPT-5", as the author believes a ".5" release may not make sense given the intense competition and scrutiny.
- The article also discusses the "GPT brand trap", where OpenAI has heavily associated its products with the GPT acronym, which may become a constraint as the technology evolves beyond the original GPT architecture.
2. How does the author analyze the competitive landscape and its impact on the GPT-5 release?
- The author argues that the gap between OpenAI and its competitors (Google, Anthropic, Meta) is closing, with models like Gemini, Claude, and Llama reaching GPT-4-class performance.
- This suggests that OpenAI may need to maintain its edge with GPT-5, as the competition is catching up and the race for the next-generation AI models is at an impasse.
- The author believes that for each new state-of-the-art generation of models, the gap between the leader and the rest shrinks, as the top AI companies have learned how to build this technology reliably.
[02] Everything We Know About GPT-5
1. What are the key insights from the sources quoted about the release timeline and capabilities of GPT-5?
- Sam Altman, the CEO of OpenAI, has been vague about the release date of GPT-5, suggesting that OpenAI may be still deciding whether to release an intermediate "GPT-4.5" model first.
- Altman has indicated that the delta in performance between GPT-4 and GPT-5 will be similar to the delta between GPT-3 and GPT-4, implying a significant improvement.
- Other sources, such as Business Insider, have reported that GPT-5 is expected to be "materially better" than previous models, but the author expresses skepticism about the reliability of these claims.
2. How does the author analyze the potential size and scaling of GPT-5 based on the available information?
- The author estimates that GPT-5 could be significantly larger than GPT-4, potentially in the range of 7-11 trillion parameters, based on OpenAI's access to Microsoft's Azure cloud and the H100 GPUs.
- However, the author also notes that the size of the model may not be the only factor, and that OpenAI could focus on improving the architecture and training techniques to enhance performance without necessarily increasing the model size.
[03] Everything We Don't Know About GPT-5
1. What are the key algorithmic breakthroughs and capabilities that the author speculates GPT-5 may incorporate?
- Multimodality: The author expects GPT-5 to have expanded multimodal capabilities, including the ability to generate and understand video, building on OpenAI's Sora project.
- Reasoning: The author believes OpenAI is working on integrating search-based reasoning and reinforcement learning techniques into GPT-5, to address the limitations of current language models in terms of robust and generalizable reasoning.
- Agents: The author speculates that GPT-5 may be accompanied by the development of AI agent capabilities, where the model can autonomously perform tasks and interact with applications, though the author is skeptical that GPT-5 itself will be a fully-fledged AI agent.
2. How does the author analyze the challenges and trade-offs involved in improving the reliability and safety of GPT-5?
- The author argues that the unreliability and unpredictability of current language models is a significant challenge, and that while OpenAI is working on improving safety and reliability through techniques like RLHF, the author is skeptical that these approaches can fully solve the problem.
- The author suggests that the fundamental issue is the lack of mechanistic interpretability of these models, which makes it difficult to guarantee their reliability and safety, even with extensive testing and red-teaming.