
How close is AI to replacing product managers?
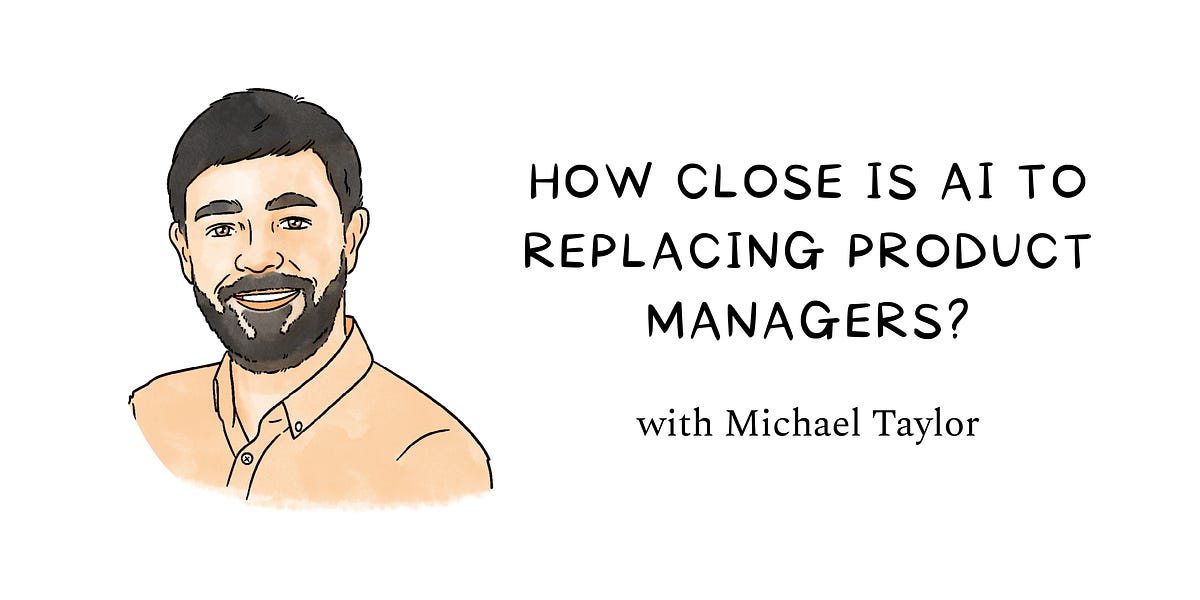
๐ Abstract
The article discusses the author's experiment to test how well AI models like ChatGPT can perform on various product management tasks compared to humans. The author, a prompt engineering expert, collaborated with Lenny Rachitsky to evaluate AI's capabilities in areas like developing product strategy, defining KPIs, and estimating feature ROI. They ran blind tests where people voted on whether the AI or human-generated responses were better, and found that the AI outperformed humans in some tasks. The article also outlines the author's prompting techniques and plans to expand the benchmark to cover more PM skills.
๐ Q&A
[01] Evaluating AI's Product Management Capabilities
1. What were the key findings from the author's experiment comparing AI and human performance on product management tasks?
- The AI answer beat the human answer in 2 out of 3 tasks tested
- 70-80% of people correctly guessed which answer was AI, but many still preferred the AI response
- There is room for improvement, as small changes to the prompts yielded better AI results
- This was just an initial test, and AI is still far from being able to independently work as a product manager
2. What were the 3 product management tasks the author selected to test? The 3 tasks were:
- Developing a product strategy
- Defining KPIs
- Estimating the ROI of a feature idea
3. How did the author approach running the blind tests to evaluate the AI and human responses?
- The author used real-world examples of human responses from Exponent's PM interview question database as the benchmark
- Lenny tweeted screenshots of the AI and human responses without revealing which was which
- People were asked to vote on which response they thought was better, and also guess which one was AI
4. What were some of the key insights the author gained from the blind test results?
- People often correctly guessed which response was AI, but still preferred the AI answer in many cases
- The AI responses tended to be more comprehensive, which was both a strength and a weakness
- Incorporating more human-like elements like obscure references and minor grammatical errors could help hide the AI origin
[02] Expanding the AI Benchmark for Product Management
1. What framework did the author propose to expand the benchmark of AI capabilities across different product management skills? The author suggested aligning the benchmark tasks with Lenny's framework for categorizing PM skills:
- Shape the product
- Ship the product
- Sync the people
2. What other ideas did the author have to make the benchmark more robust and trustworthy?
- Explore multiple questions per skill category to get more diverse results
- Test across different AI models like Claude 3.5 and Google Gemini 1.5
- Investigate how giving models access to the internet may impact their performance
- Address the issue of data contamination, where the models learn from previous benchmark results
3. What challenges did the author identify with the voting mechanism used in the initial tests?
- Posting on multiple platforms and manually tallying votes was too open to interpretation
- The author wants to find a more automated, scalable way to run the evaluations
4. What prompt engineering techniques did the author use to get better AI performance on the tasks?
- Finding real-world human examples to use as a benchmark
- Providing instructions for the AI to mimic the structure and style of the human responses
- Asking the AI to role-play as a product manager for a major tech company
- Incorporating "chain of thought" prompting to have the AI plan its response