
Bio foundation models - definitions, nuance, and tactics
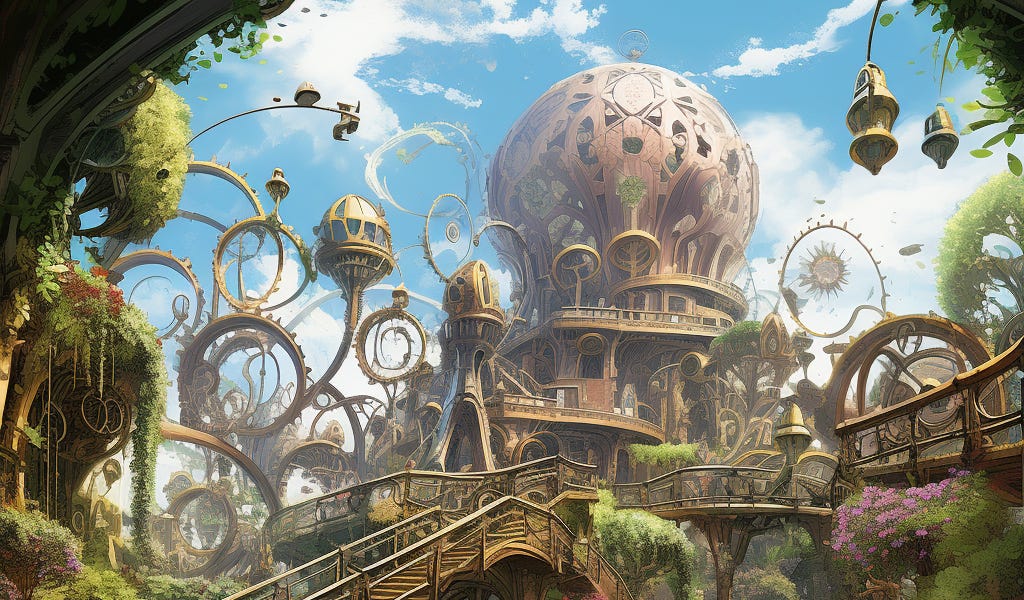
๐ Abstract
The article discusses the concept of "foundation models" in the context of biotechnology and healthcare, drawing parallels and distinctions from their usage in the tech industry. It covers the technical specifications, sociological impact, and commercialization strategies of these models across different domains such as protein structure prediction, single-cell RNA sequencing, and multimodal healthcare applications.
๐ Q&A
[01] What is the definition of "foundation models" in the context of tech and how does it translate to the bio/healthcare domain?
- In tech, "foundation models" are defined by their large scale in terms of training data size, model parameters, and sociological impact, with examples like BERT, GPT-3, and PaLM.
- In bio/healthcare, similar "foundation models" have emerged, such as AlphaFold for protein structure prediction, RoboRX for drug interaction prediction, and multimodal models integrating data from DNA sequences, gene expression, and other biological modalities.
- The sociological impact in bio/healthcare is more narrow, focused on the models becoming a substrate for various downstream applications, rather than a broader shift in AI research and deployment.
[02] What are some key differences in the commercialization of foundation models between tech and bio/healthcare?
- In tech, foundation models are primarily commercialized through API-based services, where a large pool of potential customers can access the models.
- In bio/healthcare, the pool of potential customers is smaller, so the authors believe the maximum value accrual will come from companies building products (e.g., therapeutic peptides, small molecules) around their proprietary foundation models.
- Bio/healthcare companies need to have talent for both building the foundation models and deploying the findings in the real world, which is more challenging than the tech API-based model.
- The potential size of bio/healthcare companies is capped by factors like manufacturing, regulatory milestones, and sales teams, unlike the larger tech companies.
[03] What are some examples of foundation models in the bio/healthcare domain and what are the key characteristics of these models?
- Examples include AlphaFold, RoboRX, DeepNovo, EcoRNN, and various healthcare-focused models like Med-PaLM, CLaM, FEMR, ClinicalBERT, ehrBERT, and bioGPT.
- These models often incorporate multimodal data (e.g., medical images, clinical text, structured data) and adapt training strategies and architectures from successful NLP models.
- The minimum size for foundation models in bio/healthcare seems to be around 100M parameters and 5M cells for single-cell RNA sequencing data, although some models like xTrimoPGLM have reached 100BN parameters and 1TN training tokens.
- There is a trend towards multimodal bio/healthcare foundation models, as the complexity of the domain requires integrating data from various sources.
[04] What are some unique characteristics of foundation models in the bio/healthcare domain compared to tech?
- In some areas of bio, such as protein structure prediction, surprisingly small amounts of data (e.g., 1,000 protein structures) can lead to high-performing models, suggesting the "tacit laws" of protein folding may be easier to learn than the complexities of human language.
- This means that the capital requirements for developing some bio/healthcare foundation models can be much lower (hundreds of millions) compared to the billions needed for large language models in tech.
- Companies in bio/healthcare are investing in specialized hardware and data generation (e.g., cryo-electron microscopy for protein structures) to build proprietary data moats, which is a different strategy from the tech industry.
Shared by Daniel Chen ยท
ยฉ 2024 NewMotor Inc.